
Bonferroni Test
The Bonferroni test is a type of multiple comparison test used in statistical analysis. When performing a hypothesis test with multiple comparisons, eventually a result could occur that appears to demonstrate statistical significance in the dependent variable, even when there is none. If a particular test, such as a linear regression, thus yields correct results 99% of the time, running the same regression on 100 different samples could lead to at least one false positive result at some point. This is formally called a Type I error, and as a result, an error rate that reflects the likelihood of a Type I error is assigned to the test. Using the 5% error rate from our example, two tests would yield an error rate of 0.025 or (.05/2) while four tests would therefore have an error rate of .0125 or (.05/4). The Bonferroni test is a statistical test used to reduce the instance of a false positive.
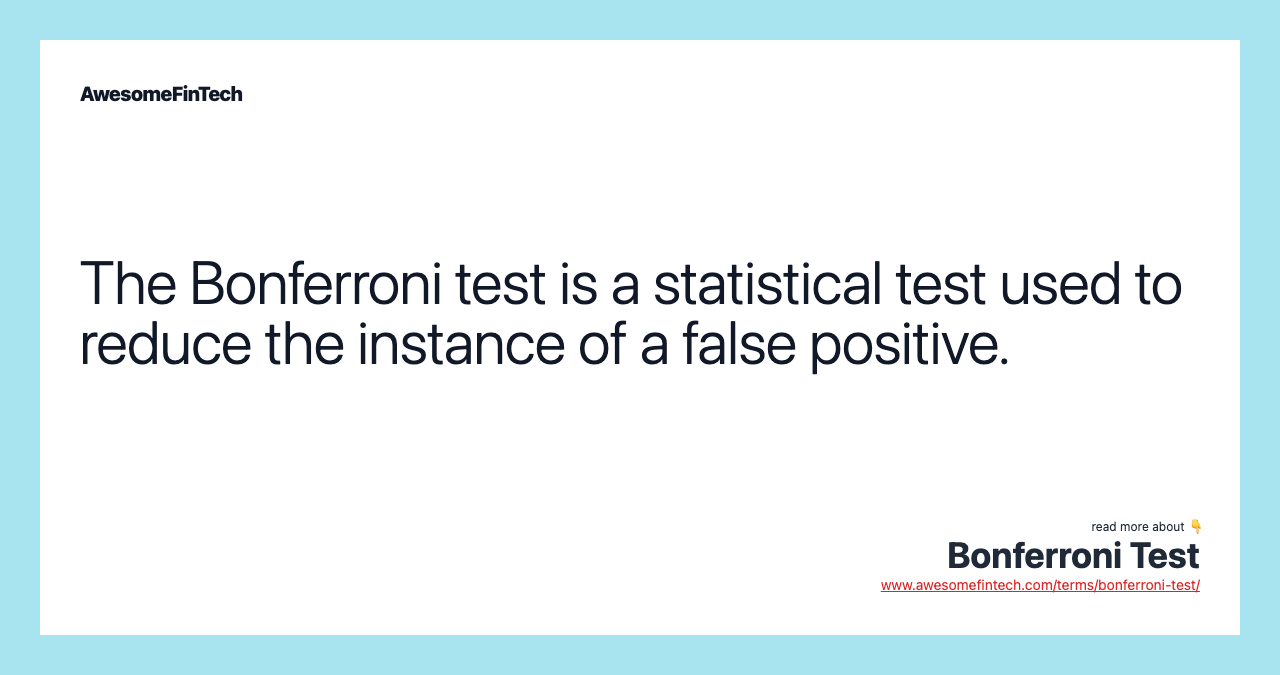
What Is the Bonferroni Test?
The Bonferroni test is a type of multiple comparison test used in statistical analysis. When performing a hypothesis test with multiple comparisons, eventually a result could occur that appears to demonstrate statistical significance in the dependent variable, even when there is none.
If a particular test, such as a linear regression, thus yields correct results 99% of the time, running the same regression on 100 different samples could lead to at least one false positive result at some point. The Bonferroni test attempts to prevent data from incorrectly appearing to be statistically significant like this by making an adjustment during comparison testing.
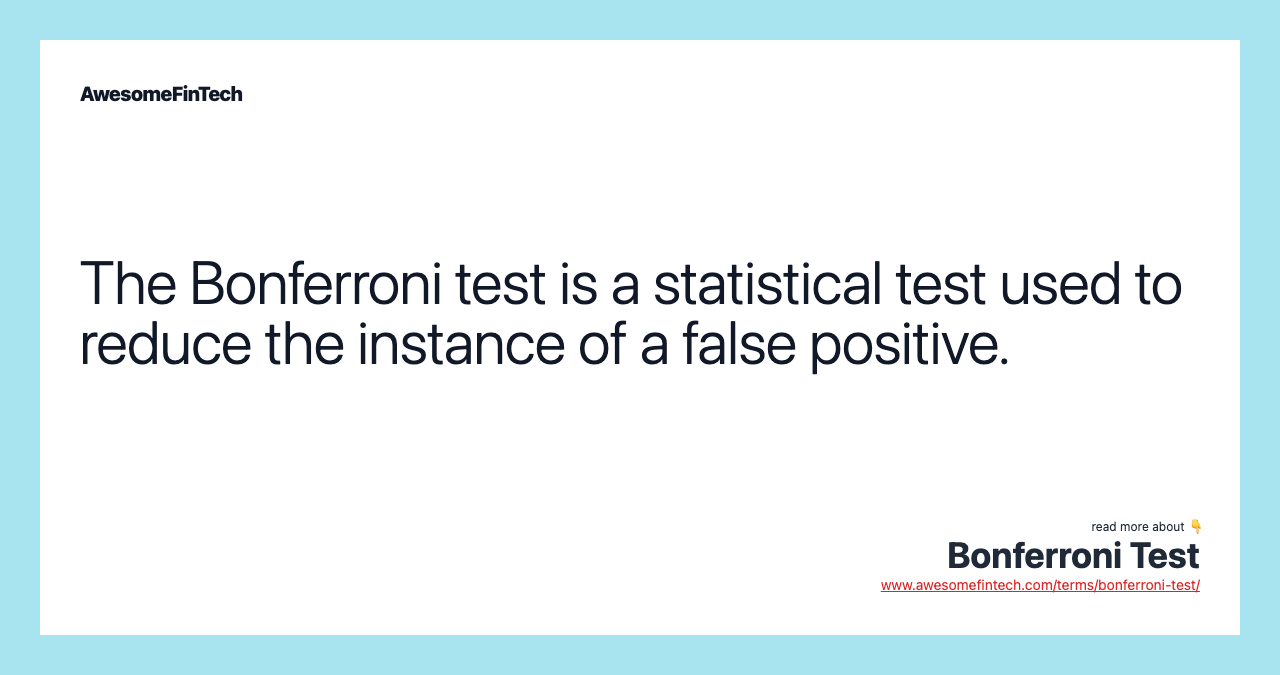
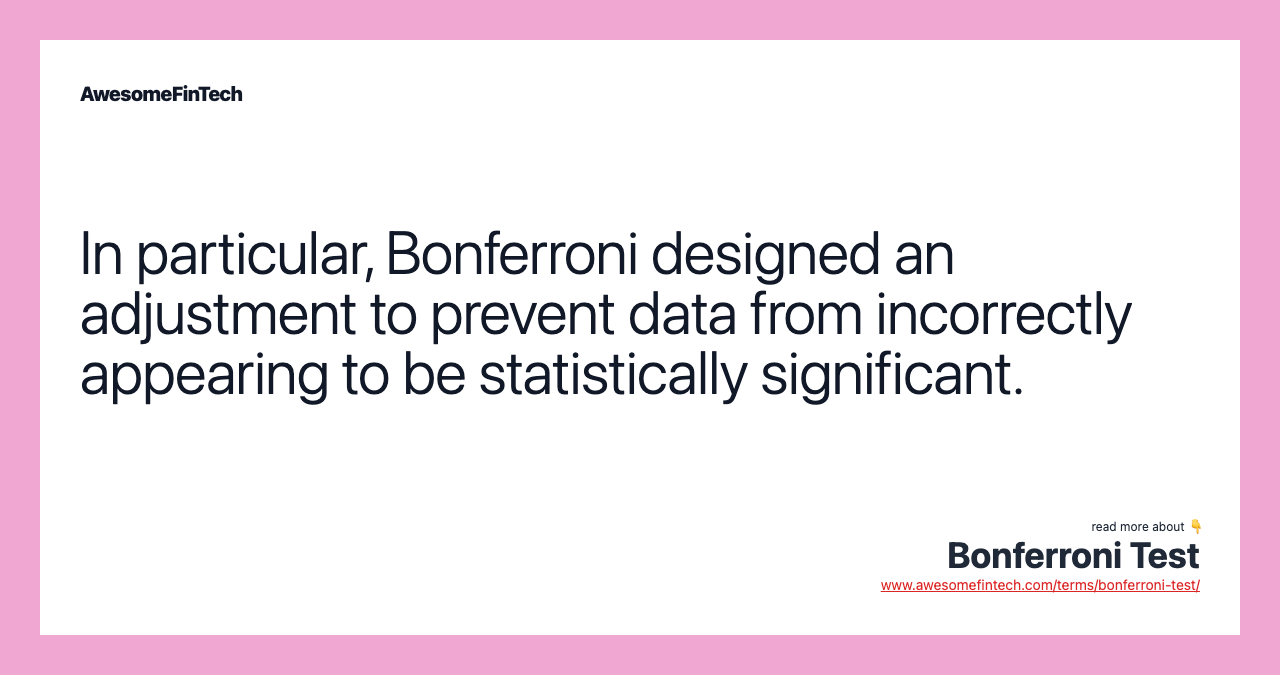
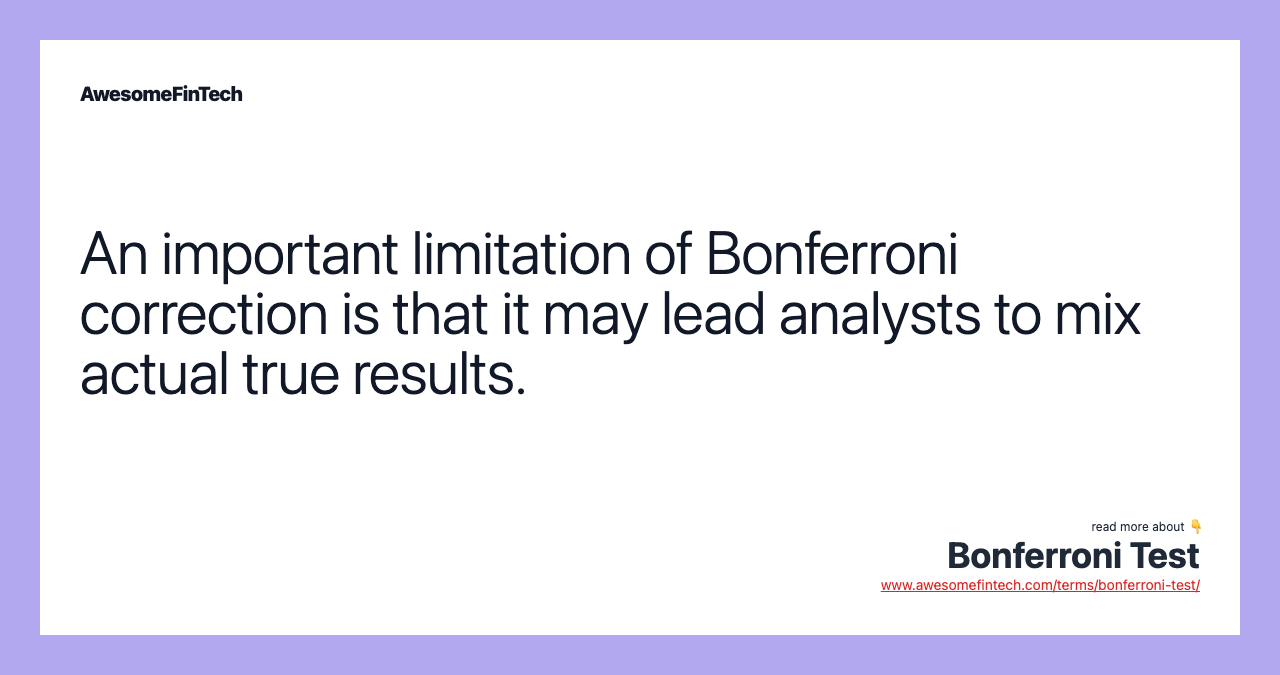
Understanding the Bonferroni Test
The Bonferroni test, also known as "Bonferroni correction" or "Bonferroni adjustment" suggests that the p-value for each test must be equal to its alpha divided by the number of tests performed.
The test is named for the Italian mathematician who developed it, Carlo Emilio Bonferroni (1892–1960). Other types of multiple comparison tests include Scheffé's test and the Tukey-Kramer method test. A criticism of the Bonferroni test is that it is too conservative and may fail to catch some significant findings.
In statistics, a null hypothesis is essentially the belief that there's no statistical difference between two data sets being compared. Hypothesis testing involves testing a statistical sample to confirm or reject a null hypothesis. The test is performed by taking a random sample of a population or group. While the null hypothesis is tested, the alternative hypothesis is also tested, whereby the two results are mutually exclusive.
However, with any testing of a null hypothesis, there's the expectation that a false positive result could occur. This is formally called a Type I error, and as a result, an error rate that reflects the likelihood of a Type I error is assigned to the test. In other words, a certain percentage of the results will likely yield a false positive.
Using Bonferroni Correction
For example, an error rate of 5% might typically be assigned to a statistical test, meaning that 5% of the time there will likely be a false positive. This 5% error rate is called the alpha level. However, when many comparisons are being made in an analysis, the error rate for each comparison can impact the other results, creating multiple false positives.
Bonferroni designed his method of correcting for the increased error rates in hypothesis testing that had multiple comparisons. Bonferroni's adjustment is calculated by taking the number of tests and dividing it into the alpha value. Using the 5% error rate from our example, two tests would yield an error rate of 0.025 or (.05/2) while four tests would therefore have an error rate of .0125 or (.05/4).
Related terms:
Alpha Risk
Alpha risk is the risk in a statistical test of rejecting a null hypothesis when it is actually true. read more
Beta Risk
Beta risk is the probability that a false null hypothesis will be accepted by a statistical test. read more
Goodness-of-Fit
A goodness-of-fit test helps you see if your sample data is accurate or somehow skewed. Discover how the popular chi-square goodness-of-fit test works. read more
Hypothesis Testing
Hypothesis testing is the process that an analyst uses to test a statistical hypothesis. The methodology employed by the analyst depends on the nature of the data used and the reason for the analysis. read more
Null Hypothesis : Testing & Examples
A null hypothesis is a type of hypothesis used in statistics that proposes that no statistical significance exists in a set of given observations. read more
P-Value
P-value is the level of marginal significance within a statistical hypothesis test, representing the probability of the occurrence of a given event. read more
Scheffé Test
A Scheffé test is a statistical test that is post-hoc test used in statistical analysis. It was named after American statistician Henry Scheffé. read more
Statistical Significance
Statistical significance refers to a result that is not likely to occur randomly but rather is likely to be attributable to a specific cause. read more
Test
A test is when a stock’s price approaches an established support or resistance level set by the market. read more
Type II Error
A type II error is a statistical term referring to the acceptance (non-rejection) of a false null hypothesis. read more