
Beta Risk
Beta risk is the probability that a false null hypothesis will be accepted by a statistical test. Beta risk contrasts with alpha risk, which measures the probability that a null hypothesis is rejected when it is actually true. Beta risk may be defined as the risk found in incorrectly accepting the null hypothesis when an alternative hypothesis is true. Beta, which is part of the capital asset pricing model and measures the relative volatility of a security, is only remotely related to beta risk in decision-making. A statistical test should be employed to detect differences and the beta risk is the probability that a statistical test will be unable to do so.
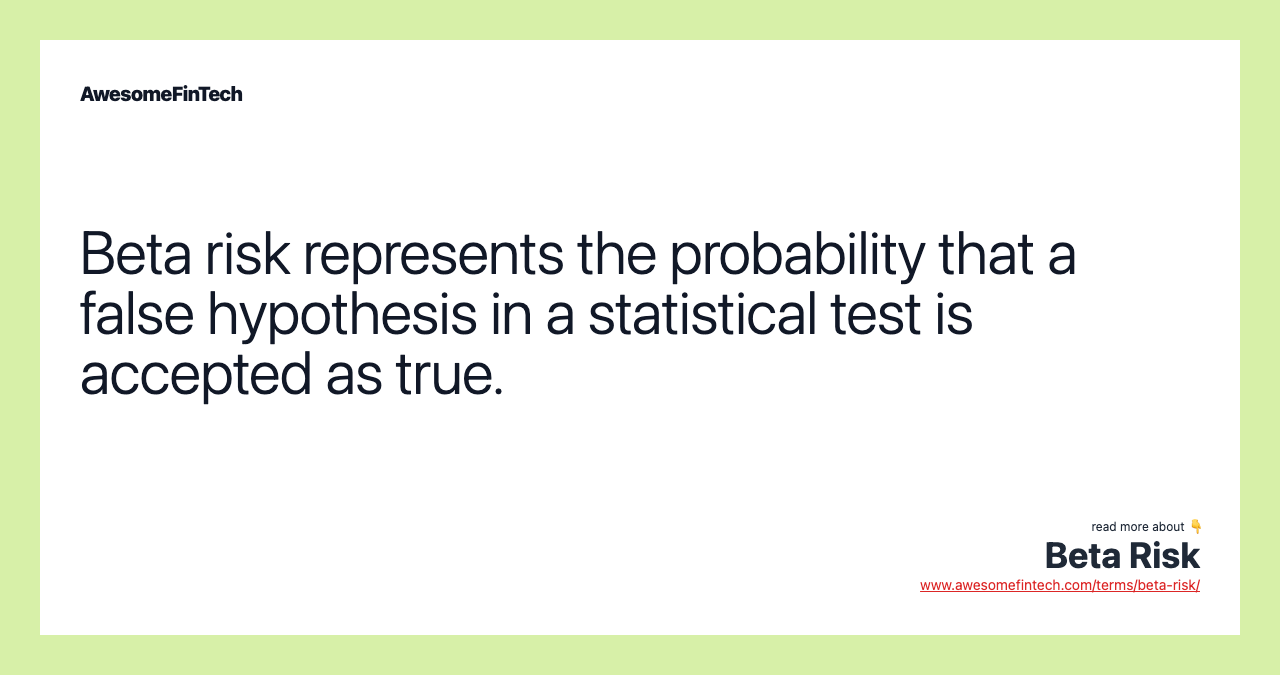
What Is Beta Risk?
Beta risk is the probability that a false null hypothesis will be accepted by a statistical test. This is also known as a Type II error or consumer risk. In this context, the term "risk" refers to the chance or likelihood of making an incorrect decision. The primary determinant of the amount of beta risk is the sample size used for the test. Specifically, the larger the sample tested, the lower the beta risk becomes.
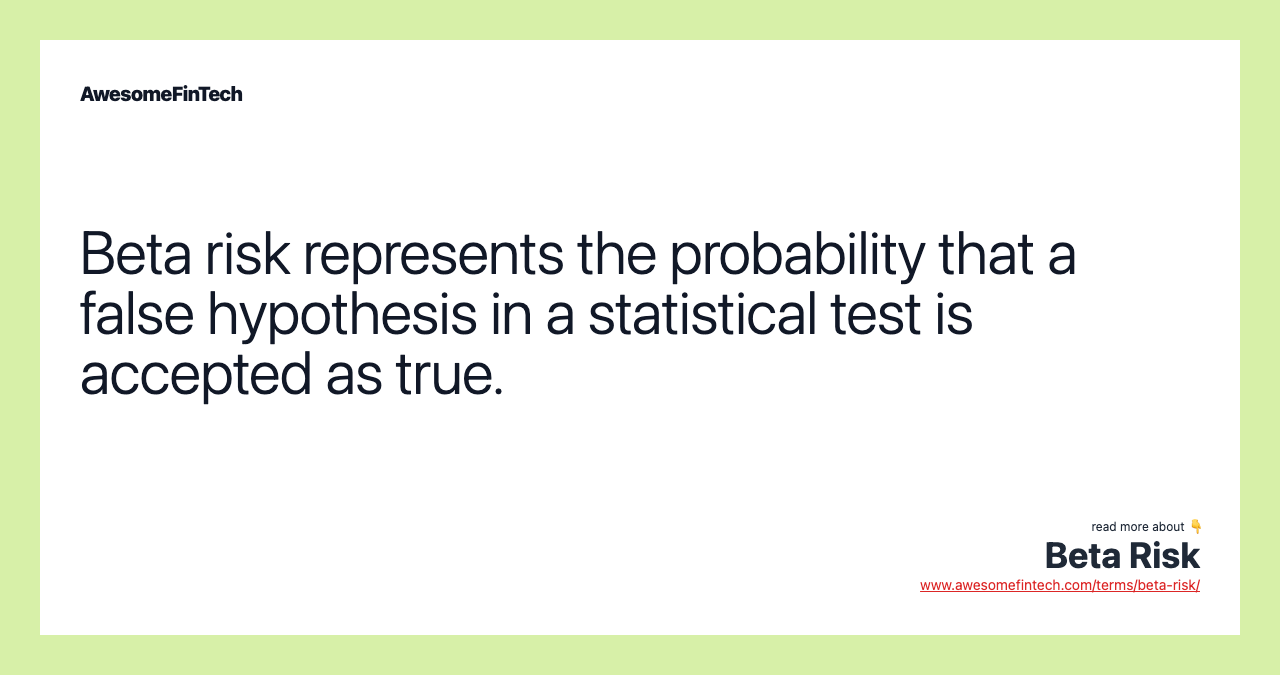
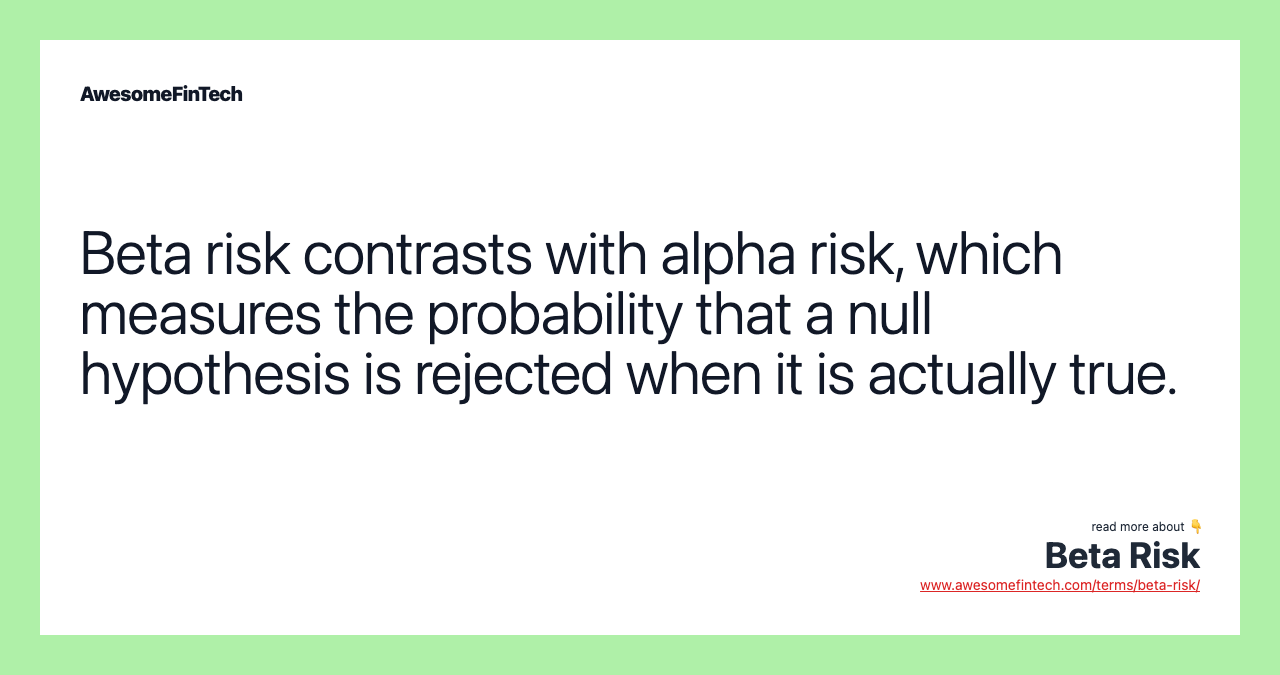
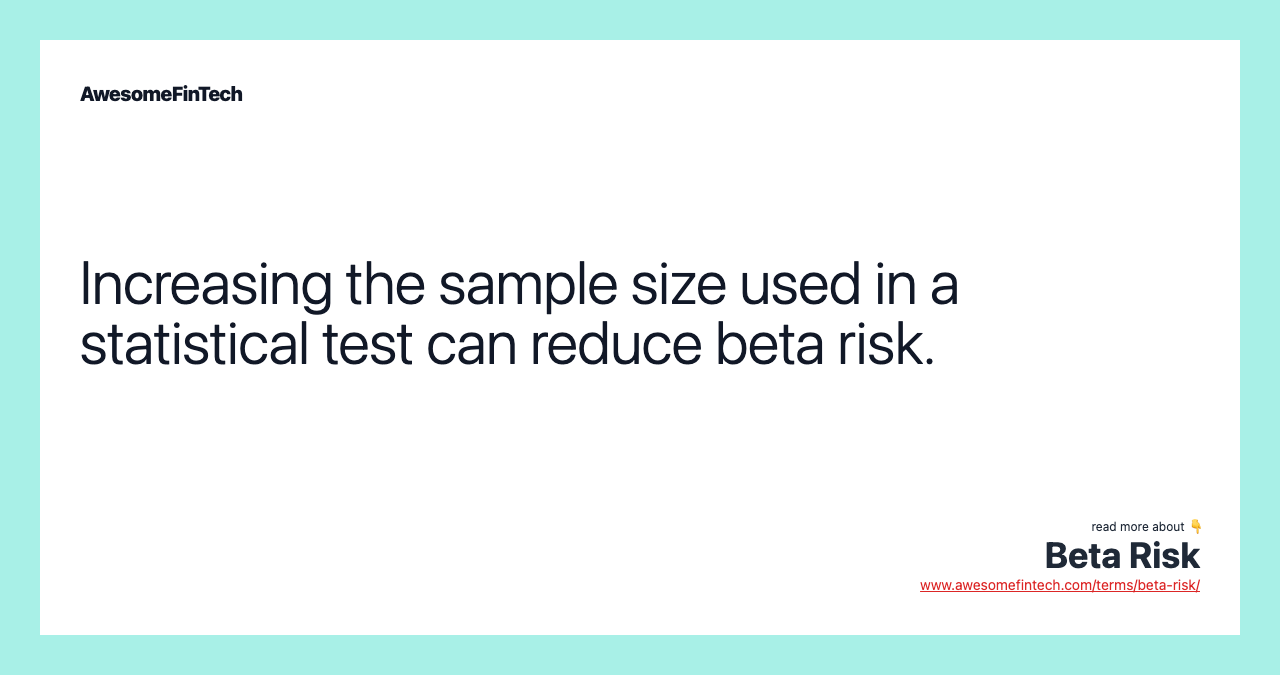
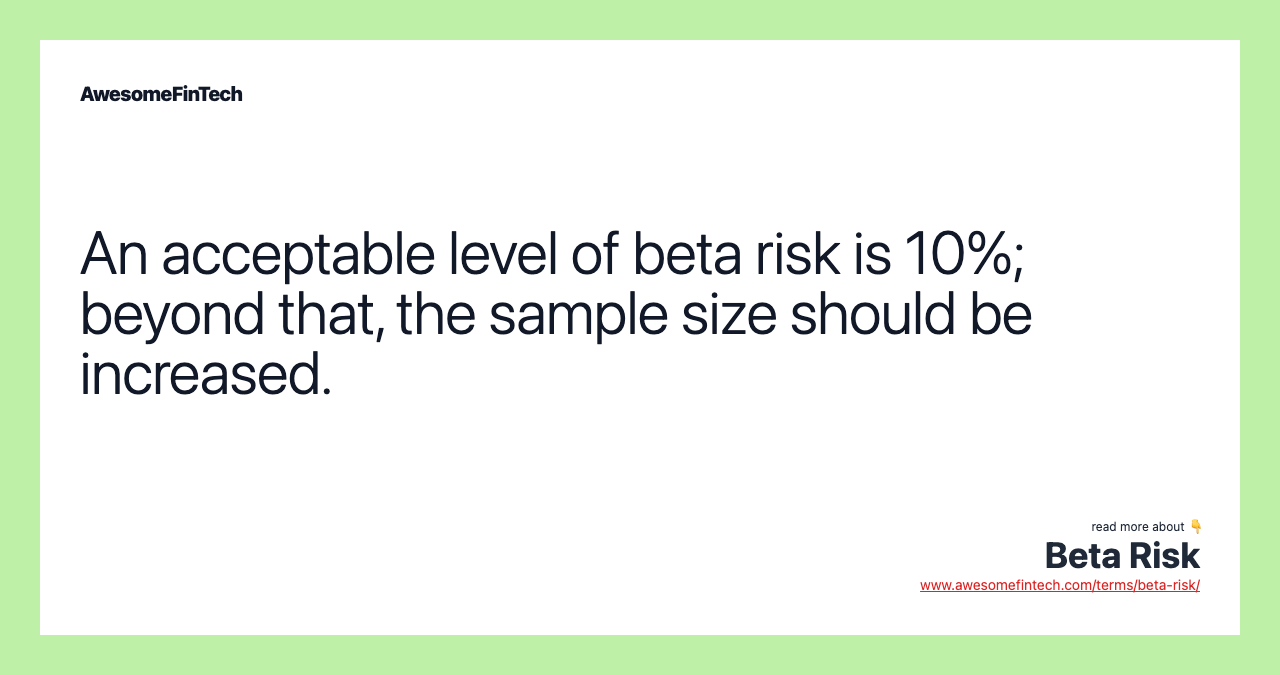
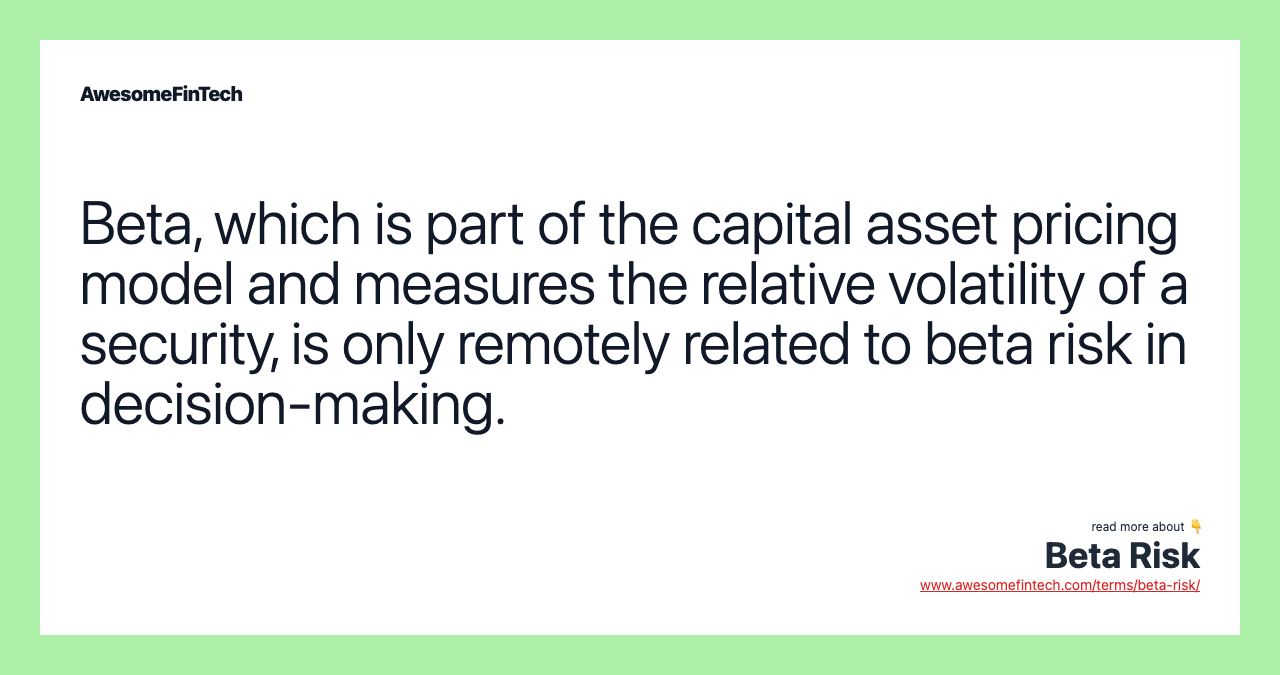
Understanding Beta Risk
Beta risk may be defined as the risk found in incorrectly accepting the null hypothesis when an alternative hypothesis is true. Put simply, it is taking the position that there is no difference when, in fact, there is one. A statistical test should be employed to detect differences and the beta risk is the probability that a statistical test will be unable to do so. For example, if beta risk is 0.05, there is a 5% likelihood of inaccuracy.
Beta risk is sometimes called "beta error" and is often paired with "alpha risk," also known as a Type I error. Alpha risk is an error occurring when a null hypothesis is rejected when it is actually true. It is also known as "producer risk." The best way to decrease alpha risk is to increase the size of the sample being tested with the hope that the larger sample will be more representative of the population.
Beta risk is based on the characteristics and nature of a decision that is being taken and may be determined by a company or individual. It depends on the magnitude of the variance between sample means. The way to manage beta risk is by boosting the test sample size. An acceptable level of beta risk in decision-making is about 10%. Any number higher should trigger increasing the sample size.
Examples of Beta Risk
An interesting application of hypothesis testing in finance can be made using the Altman Z-score. The Z-score is a statistical model meant to predict the future bankruptcy of firms based on certain financial indicators.
Statistical tests of the accuracy of the Z-score have indicated relatively high accuracy, predicting bankruptcy within one year. These tests show a beta risk (firms predicted to go bankrupt but did not) ranging from approximately 15% to 20%, depending on the sample being tested.
In 2007, Altman Z-score indicated that the companies' risks were increasing significantly as the credit ratings of specific asset-related securities had been rated higher than they should have been. The median Altman Z-score of companies in 2007 was 1.81, which is very close to the threshold that would indicate a high probability of becoming bankrupt; Altman's calculations led him to believe a crisis would occur.
The Z-score should be calculated and interpreted with care. For example, the Z-score is not immune to false accounting practices. Since companies in trouble may sometimes misrepresent or cover up their financials, the Z-score is only as accurate as the data that goes into it.
Beta Risk vs. Beta
Beta, in the context of investing, is also known as beta coefficient and is a measure of the volatility, or systematic risk, of a security or a portfolio in comparison to the market as a whole. In short, the beta of an investment indicated whether it is more or less volatile compared to the market.
It is a component of the capital asset pricing model (CAPM), which calculates the expected return of an asset based on its beta and expected market returns. As such, beta is only tangentially related to beta risk in the context of decision-making.
Related terms:
Alpha Risk
Alpha risk is the risk in a statistical test of rejecting a null hypothesis when it is actually true. read more
Altman Z-Score
The Altman Z-score is the output of a credit-strength test that gauges a publicly traded manufacturing company's likelihood of bankruptcy. read more
Capital Asset Pricing Model (CAPM)
The Capital Asset Pricing Model is a model that describes the relationship between risk and expected return. read more
Creative Accounting
Creative accounting consists of accounting practices that follow required laws and regulations, but deviate from what those standards intend to accomplish. read more
Goodness-of-Fit
A goodness-of-fit test helps you see if your sample data is accurate or somehow skewed. Discover how the popular chi-square goodness-of-fit test works. read more
Hypothesis Testing
Hypothesis testing is the process that an analyst uses to test a statistical hypothesis. The methodology employed by the analyst depends on the nature of the data used and the reason for the analysis. read more
Null Hypothesis : Testing & Examples
A null hypothesis is a type of hypothesis used in statistics that proposes that no statistical significance exists in a set of given observations. read more
Sample
A sample is a smaller, manageable version of a larger group. Samples are used in statistical testing when population sizes are too large. read more
T-Test
A t-test is a type of inferential statistic used to determine if there is a significant difference between the means of two groups, which may be related in certain features. read more
Two-Tailed Test
A two-tailed test is the statistical testing of whether a distribution is two-sided and if a sample is greater than or less than a range of values. read more