
Autocorrelation
Table of Contents What Is Autocorrelation? Understanding Autocorrelation Autocorrelation in Technical Analysis Example of Autocorrelation Day % Gain or Loss Next Day's % Gain or Loss When calculating autocorrelation, the result can range from -1 to +1. An autocorrelation of +1 represents a perfect positive correlation (an increase seen in one time series leads to a proportionate increase in the other time series). Values closer to 0 indicate a greater degree of positive correlation, values closer to 4 indicate a greater degree of negative autocorrelation, while values closer to the middle suggest less autocorrelation.  On the other hand, an autocorrelation of -1 represents a perfect negative correlation (an increase seen in one time series results in a proportionate decrease in the other time series). It's conceptually similar to the correlation between two different time series, but autocorrelation uses the same time series twice: once in its original form and once lagged one or more time periods.
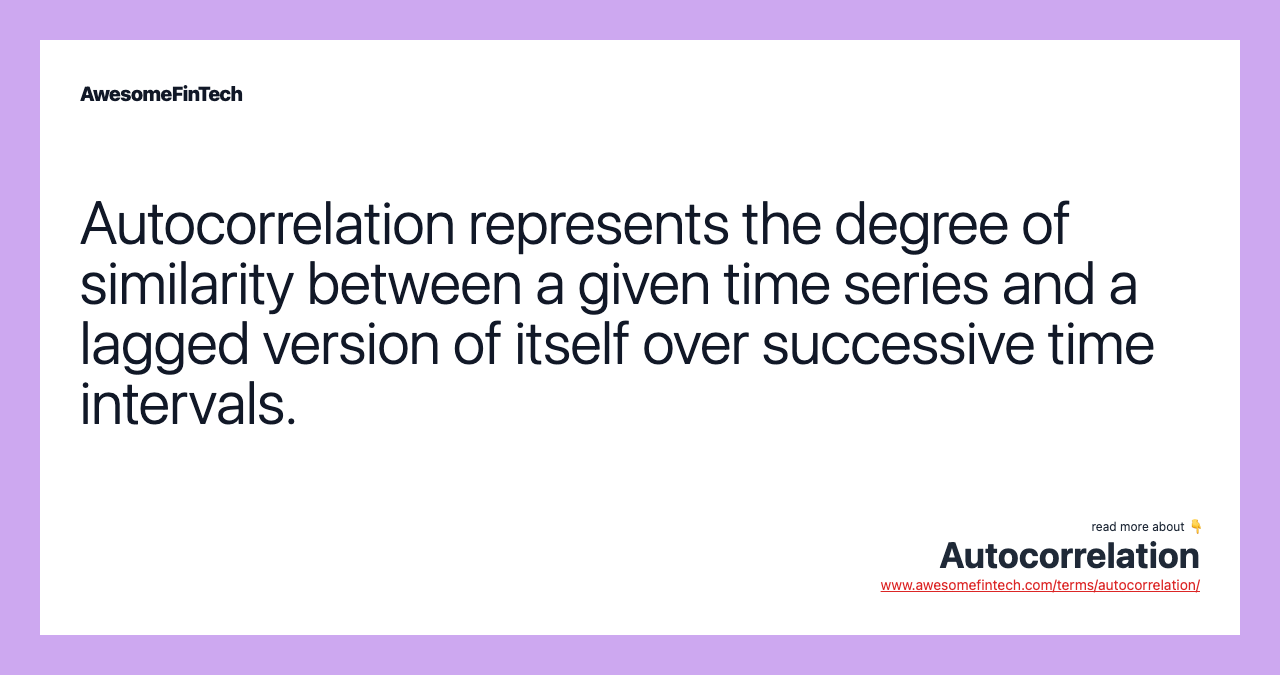
What Is Autocorrelation?
Autocorrelation is a mathematical representation of the degree of similarity between a given time series and a lagged version of itself over successive time intervals. It's conceptually similar to the correlation between two different time series, but autocorrelation uses the same time series twice: once in its original form and once lagged one or more time periods.
For example, if it's rainy today, the data suggests that it's more likely to rain tomorrow than if it's clear today. When it comes to investing, a stock might have a strong positive autocorrelation of returns, suggesting that if it's "up" today, it's more likely to be up tomorrow, too.
Naturally, autocorrelation can be a useful tool for traders to utilize; particularly for technical analysts.
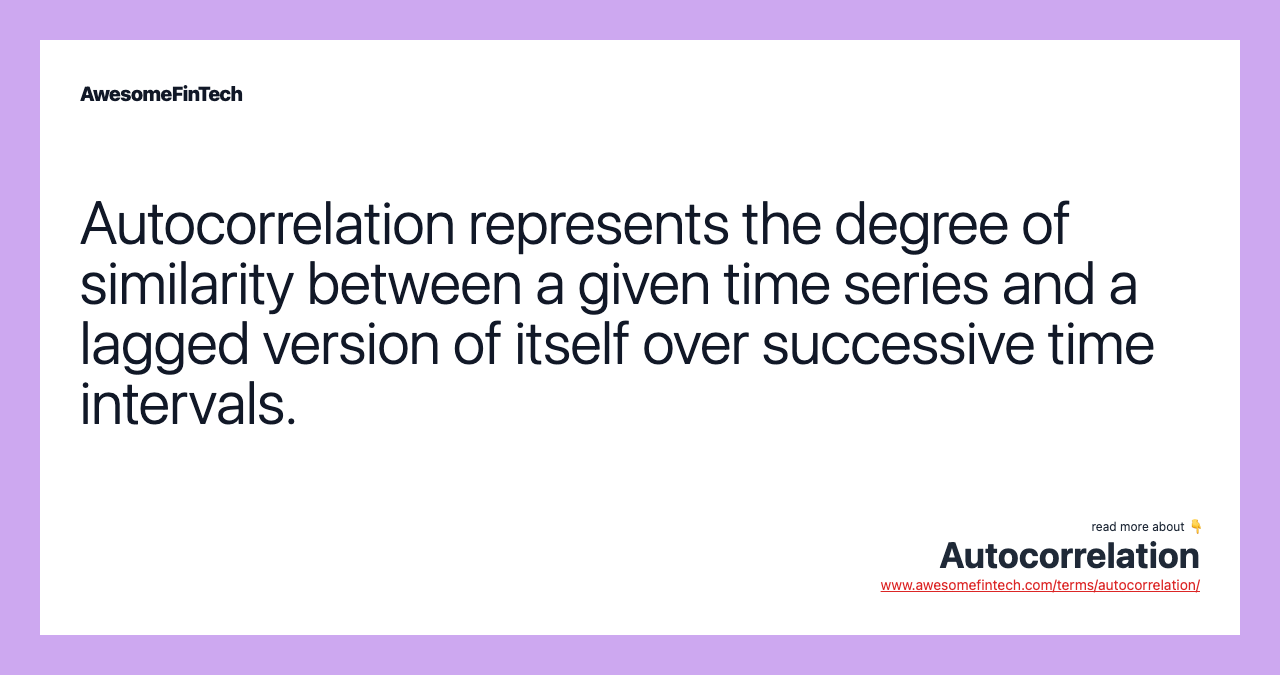
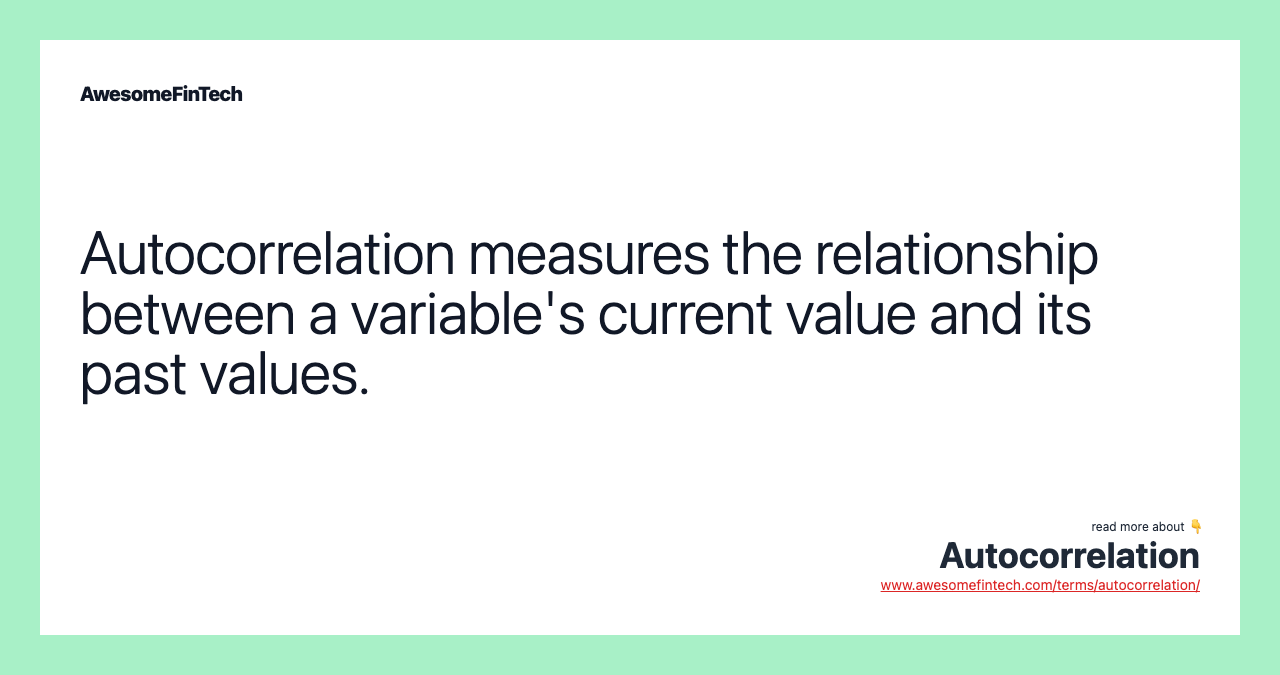
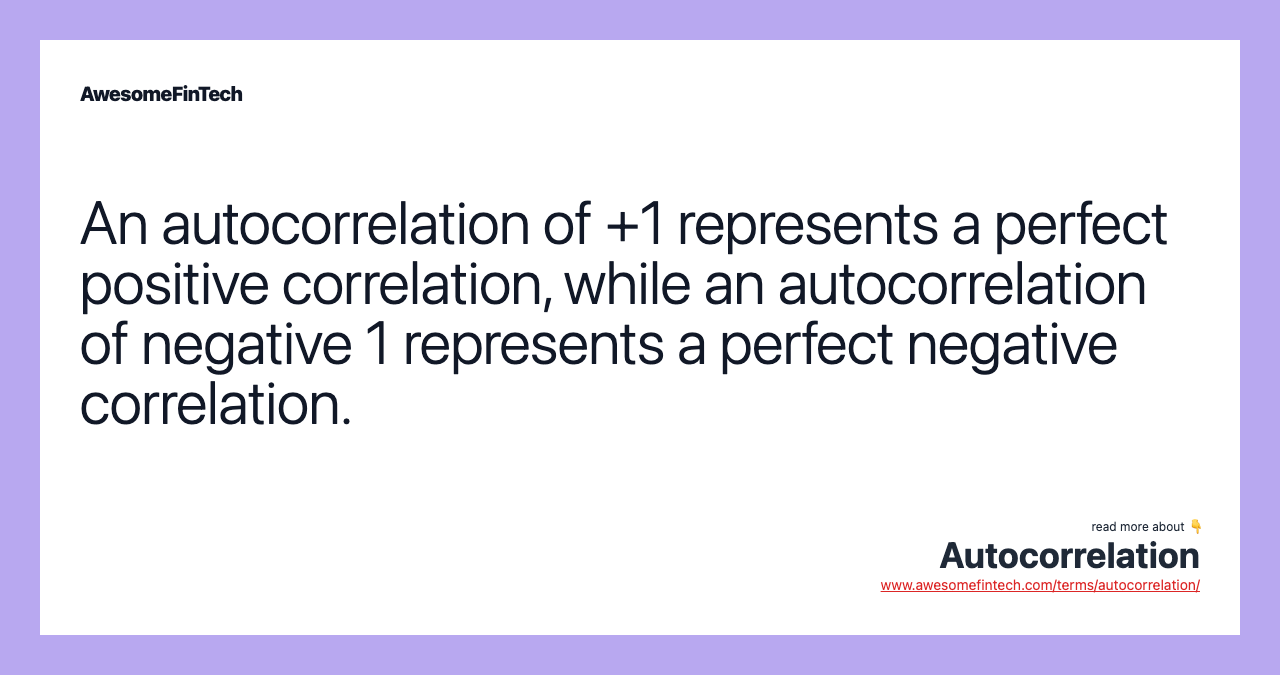
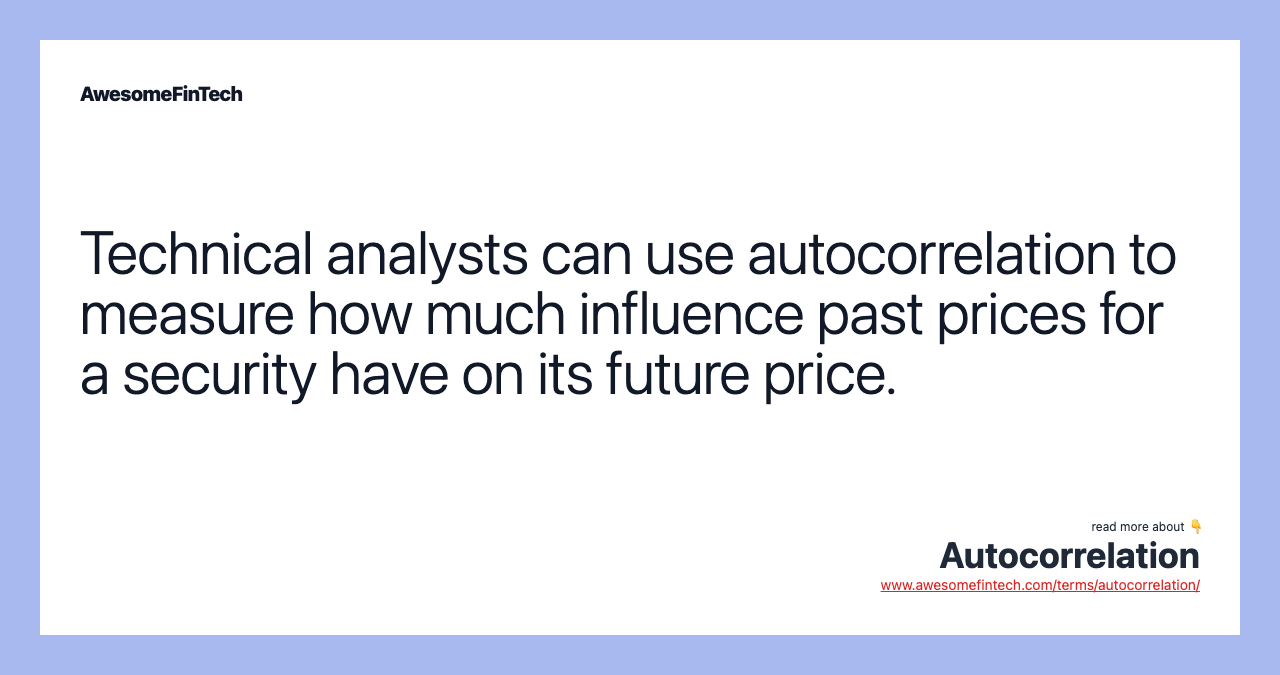
Understanding Autocorrelation
Autocorrelation can also be referred to as lagged correlation or serial correlation, as it measures the relationship between a variable's current value and its past values.
As a very simple example, take a look at the five percentage values in the chart below. We are comparing them to the column on the right, which contains the same set of values, just moved up one row.
% Gain or Loss
Next Day's % Gain or Loss
When calculating autocorrelation, the result can range from -1 to +1.
An autocorrelation of +1 represents a perfect positive correlation (an increase seen in one time series leads to a proportionate increase in the other time series).
On the other hand, an autocorrelation of -1 represents a perfect negative correlation (an increase seen in one time series results in a proportionate decrease in the other time series).
Autocorrelation measures linear relationships. Even if the autocorrelation is minuscule, there can still be a nonlinear relationship between a time series and a lagged version of itself.
Testing for Autocorrelation
The most common method of test autocorrelation is the Durbin-Watson test. Without getting too technical, the Durbin-Watson is a statistic that detects autocorrelation from a regression analysis.
The Durbin-Watson always produces a test number range from 0 to 4. Values closer to 0 indicate a greater degree of positive correlation, values closer to 4 indicate a greater degree of negative autocorrelation, while values closer to the middle suggest less autocorrelation.
So why is autocorrelation important in financial markets? Simple. Autocorrelation can be applied to thoroughly analyze historical price movements, which investors can then use to predict future price movements. Specifically, autocorrelation can be used to determine if a momentum trading strategy makes sense.
Autocorrelation in Technical Analysis
Autocorrelation can be useful for technical analysis, That's because technical analysis is most concerned with the trends of, and relationships between, security prices using charting techniques. This is in contrast with fundamental analysis, which focuses instead on a company's financial health or management.
Technical analysts can use autocorrelation to figure out how much of an impact past prices for a security have on its future price.
Autocorrelation can help determine if there is a momentum factor at play with a given stock. If a stock with a high positive autocorrelation posts two straight days of big gains, for example, it might be reasonable to expect the stock to rise over the _next t_wo days, as well.
Example of Autocorrelation
Let’s assume Emma is looking to determine if a stock's returns in her portfolio exhibit autocorrelation; that is, the stock's returns relate to its returns in previous trading sessions.
If the returns exhibit autocorrelation, Emma could characterize it as a momentum stock because past returns seem to influence future returns. Emma runs a regression with the prior trading session's return as the independent variable and the current return as the dependent variable. She finds that returns one day prior have a positive autocorrelation of 0.8.
Since 0.8 is close to +1, past returns seem to be a very good positive predictor of future returns for this particular stock.
Therefore, Emma can adjust her portfolio to take advantage of the autocorrelation, or momentum, by continuing to hold her position or accumulating more shares.
Related terms:
Accumulation
Accumulation means increasing the size of a position. It can also refer to an asset that is heavily bought and to the growth of a portfolio over time. read more
Autoregressive Integrated Moving Average (ARIMA)
An autoregressive integrated moving average (ARIMA) is a statistical analysis model that leverages time series data to forecast future trends. read more
Coefficient of Determination: Overview
The coefficient of determination is a measure used in statistical analysis to assess how well a model explains and predicts future outcomes. read more
Correlation Coefficient
The correlation coefficient is a statistical measure that calculates the strength of the relationship between the relative movements of two variables. read more
Durbin Watson Statistic
The Durbin Watson statistic is a number that tests for autocorrelation in the residuals from a statistical regression analysis. read more
Momentum
Momentum is the rate of acceleration of a security's price or volume. Momentum generally refers to the speed of movement and is usually defined as a rate. read more
Momentum Investing
Momentum investing is a strategy that aims to capitalize on the continuance of existing trends in the market. read more
Negative Correlation
Negative correlation is a relationship between two variables in which one variable increases as the other decreases, and vice versa. read more
Portfolio
A portfolio is a collection of financial investments like stocks, bonds, commodities, cash, and cash equivalents, including mutual funds and ETFs. read more
Positive Correlation
Positive correlation is a relationship between two variables in which both variables move in tandem. read more