
Z-Test
A z-test is a statistical test used to determine whether two population means are different when the variances are known and the sample size is large. A z-score, or z-statistic, is a number representing how many standard deviations above or below the mean population the score derived from a z-test is. A z-statistic, or z-score, is a number representing how many standard deviations above or below the mean population a score derived from a z-test is. Examples of tests that can be conducted as z-tests include a one-sample location test, a two-sample location test, a paired difference test, and a maximum likelihood estimate. If the standard deviation of the population is unknown, but the sample size is greater than or equal to 30, then the assumption of the sample variance equaling the population variance is made while using the z-test.
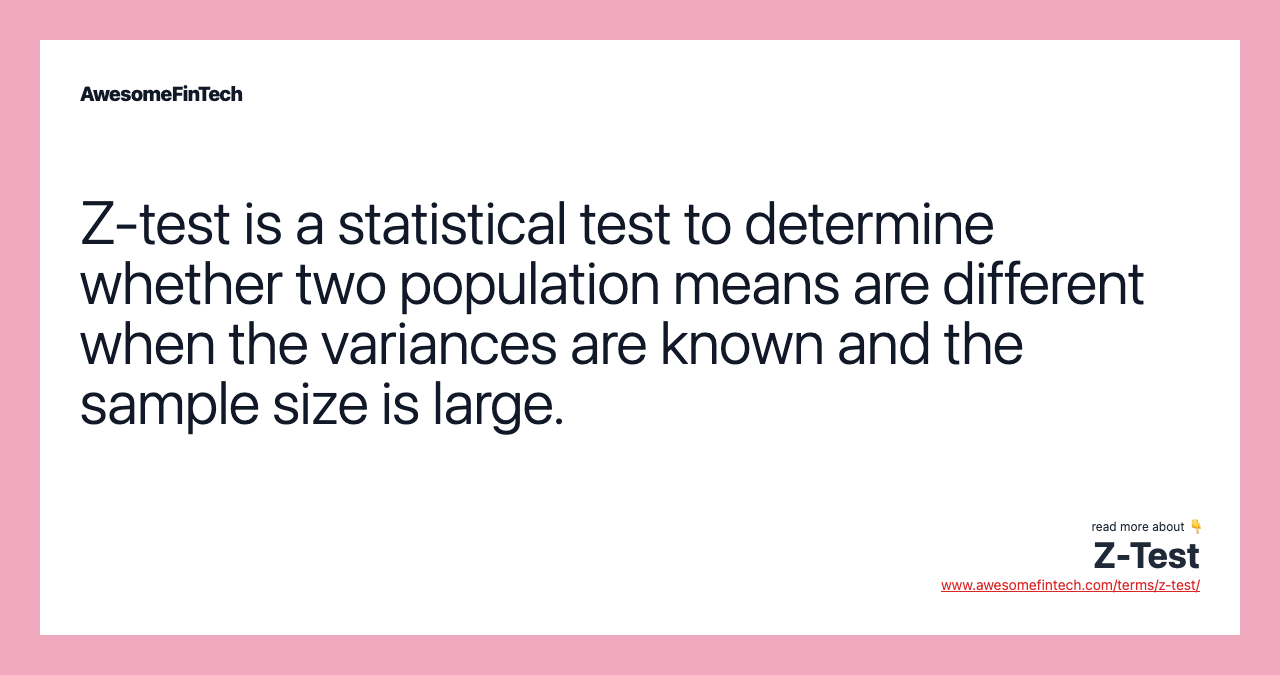
What Is a Z-Test?
A z-test is a statistical test used to determine whether two population means are different when the variances are known and the sample size is large.
The test statistic is assumed to have a normal distribution, and nuisance parameters such as standard deviation should be known in order for an accurate z-test to be performed.
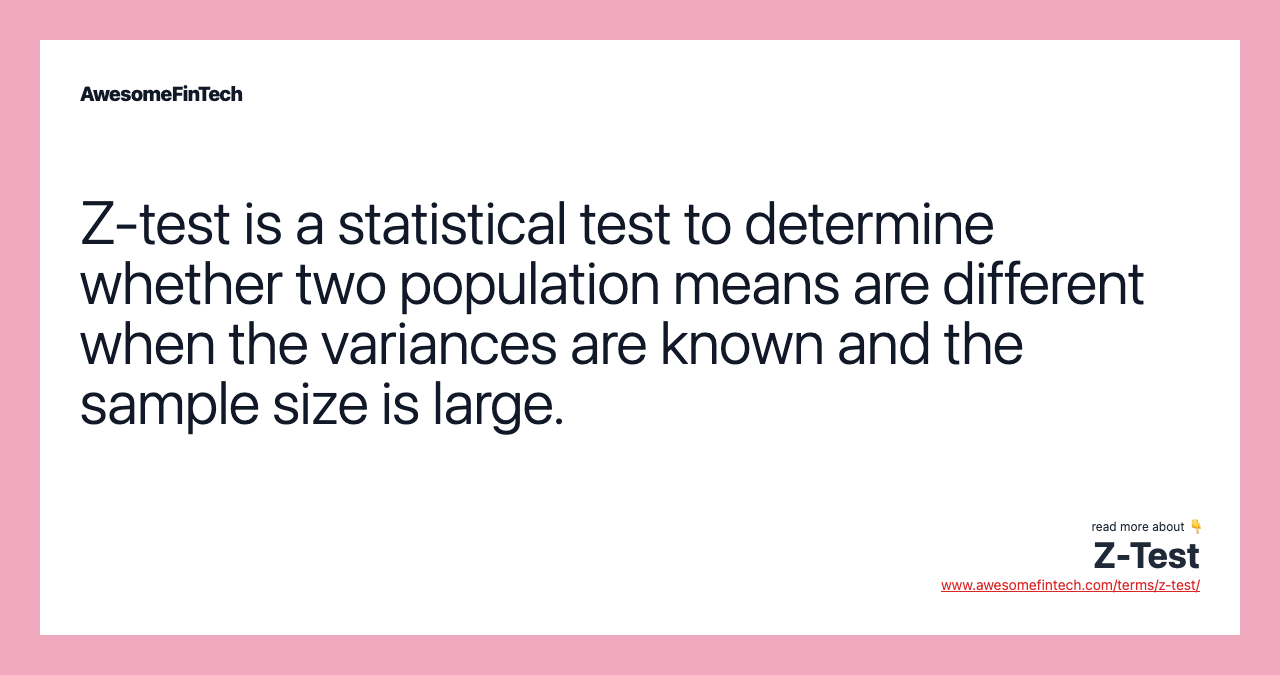
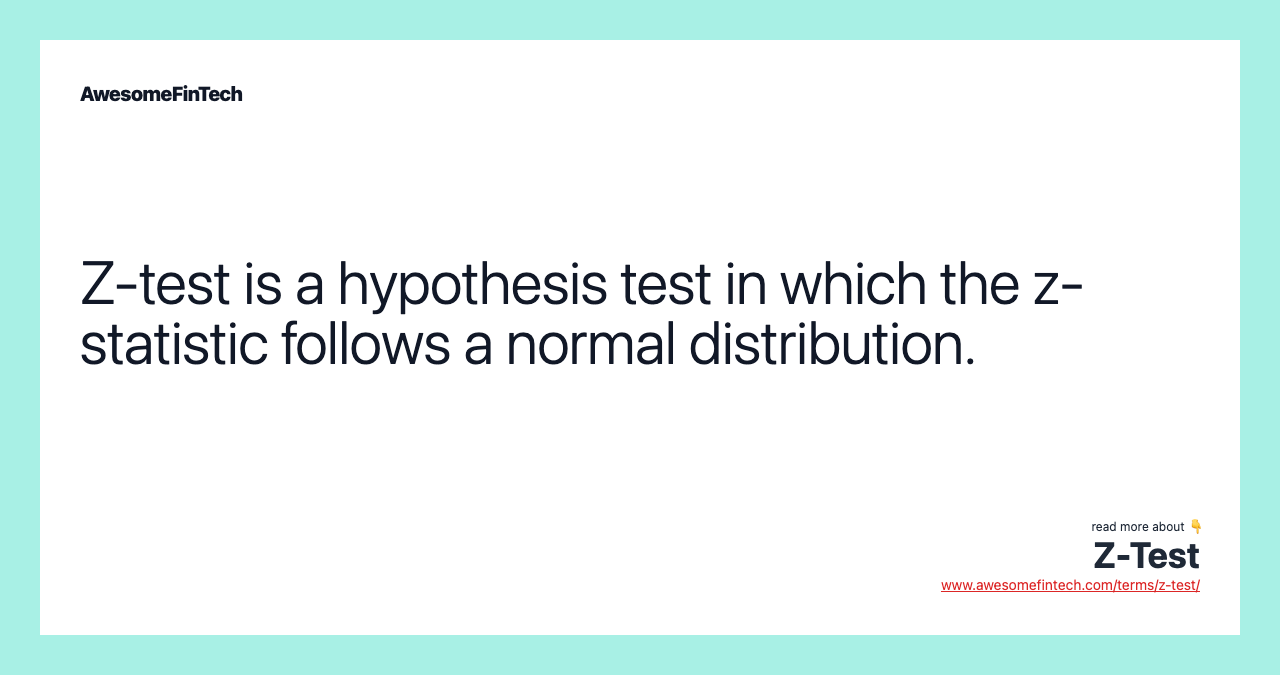
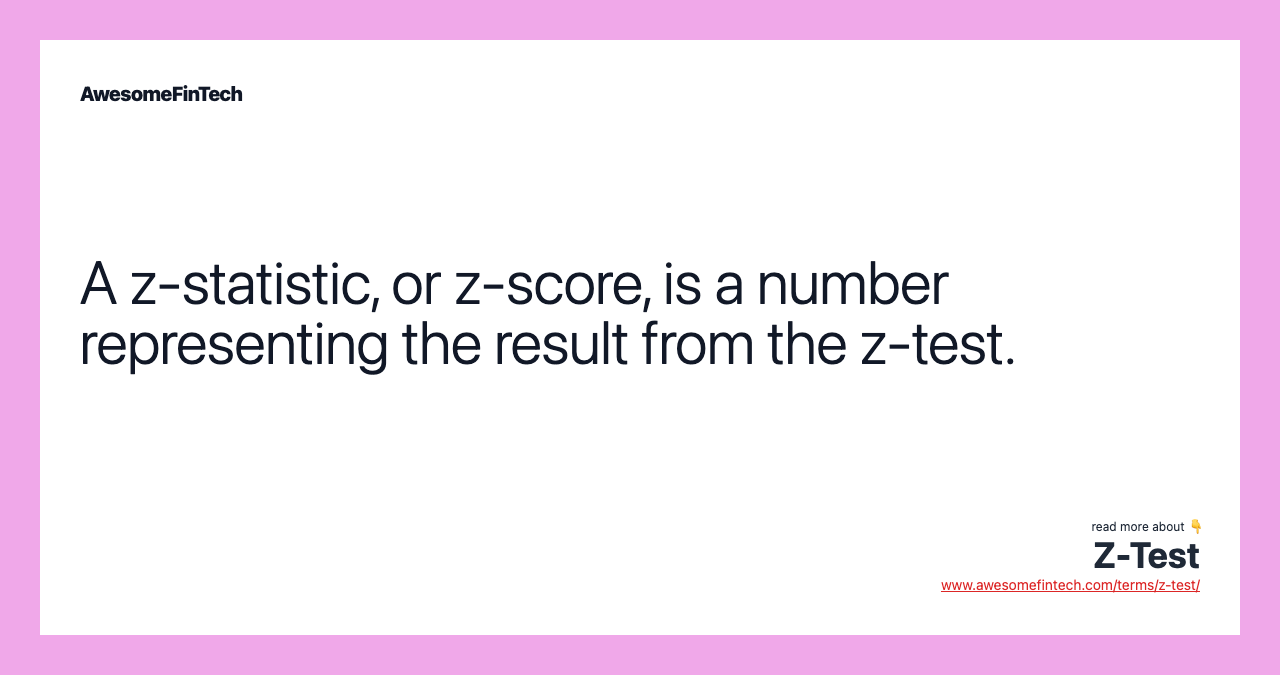
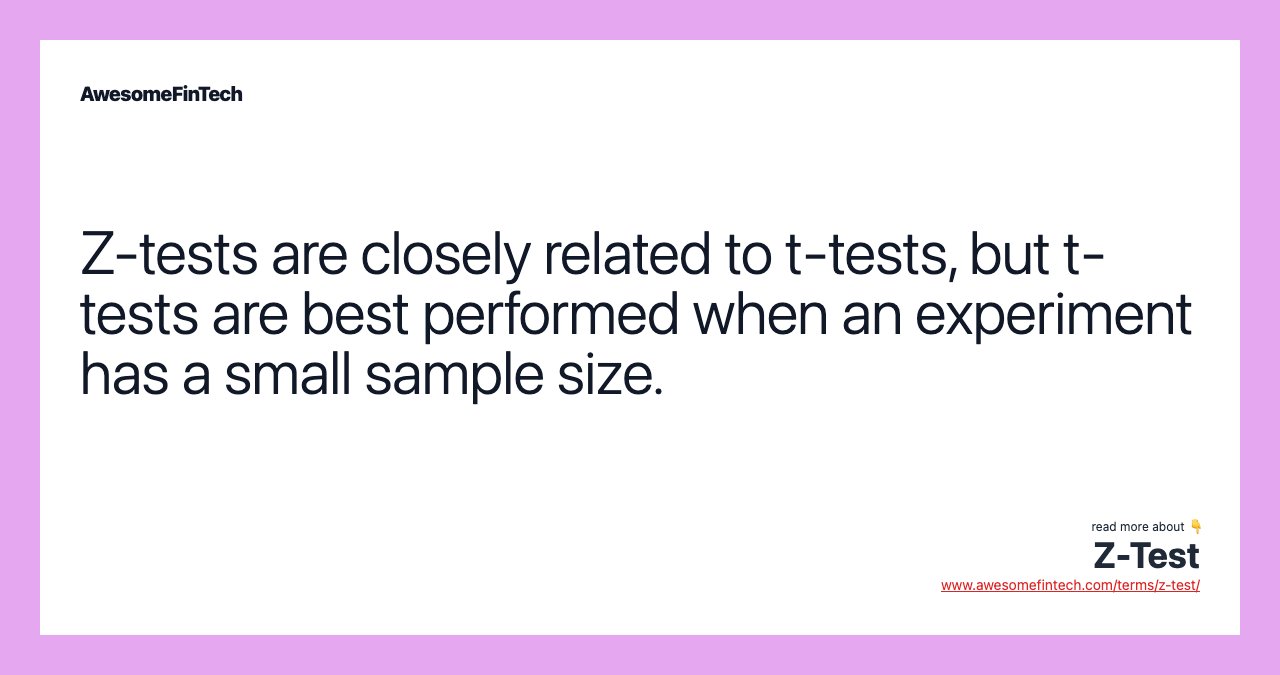
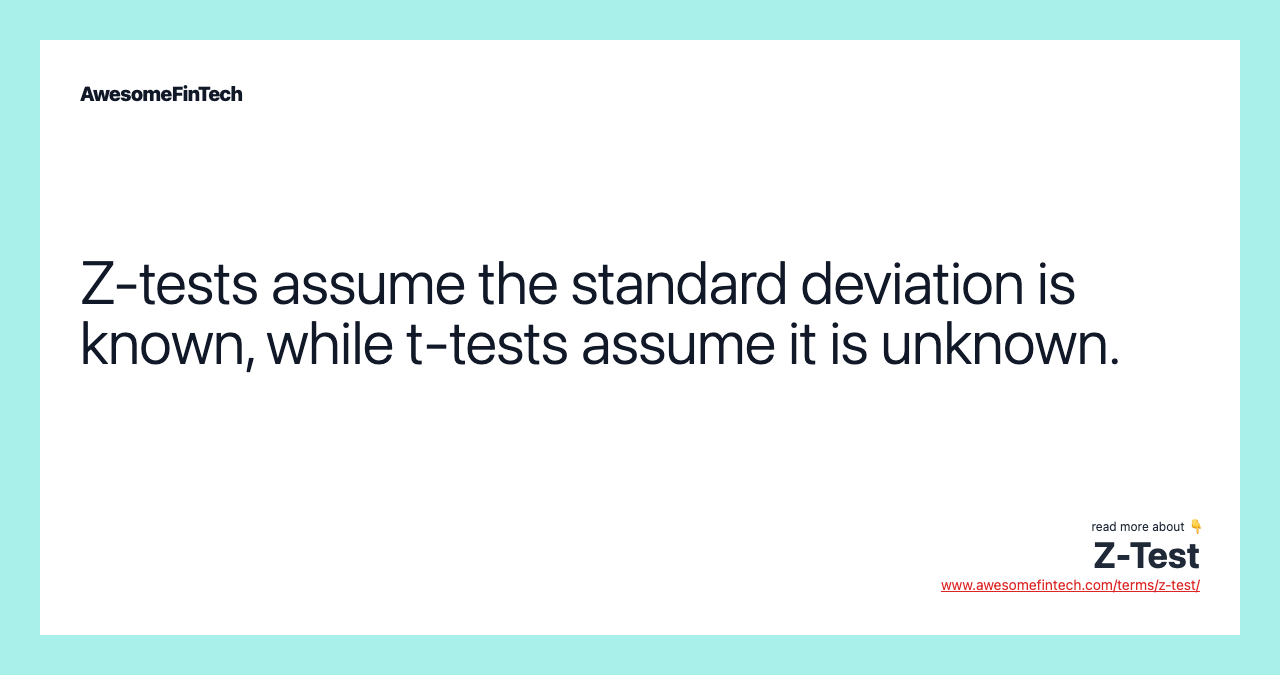
Understanding Z-Test
The z-test is also a hypothesis test in which the z-statistic follows a normal distribution. The z-test is best used for greater-than-30 samples because, under the central limit theorem, as the number of samples gets larger, the samples are considered to be approximately normally distributed.
When conducting a z-test, the null and alternative hypotheses, alpha and z-score should be stated. Next, the test statistic should be calculated, and the results and conclusion stated. A z-statistic, or z-score, is a number representing how many standard deviations above or below the mean population a score derived from a z-test is.
Examples of tests that can be conducted as z-tests include a one-sample location test, a two-sample location test, a paired difference test, and a maximum likelihood estimate. Z-tests are closely related to t-tests, but t-tests are best performed when an experiment has a small sample size. Also, t-tests assume the standard deviation is unknown, while z-tests assume it is known. If the standard deviation of the population is unknown, the assumption of the sample variance equaling the population variance is made.
One-Sample Z-Test Example
Assume an investor wishes to test whether the average daily return of a stock is greater than 3%. A simple random sample of 50 returns is calculated and has an average of 2%. Assume the standard deviation of the returns is 2.5%. Therefore, the null hypothesis is when the average, or mean, is equal to 3%.
Conversely, the alternative hypothesis is whether the mean return is greater or less than 3%. Assume an alpha of 0.05% is selected with a two-tailed test. Consequently, there is 0.025% of the samples in each tail, and the alpha has a critical value of 1.96 or -1.96. If the value of z is greater than 1.96 or less than -1.96, the null hypothesis is rejected.
The value for z is calculated by subtracting the value of the average daily return selected for the test, or 1% in this case, from the observed average of the samples. Next, divide the resulting value by the standard deviation divided by the square root of the number of observed values.
Therefore, the test statistic is:
(0.02 - 0.01) ÷ (0.025 ÷ √ 50) = 2.83
The investor rejects the null hypothesis since z is greater than 1.96 and concludes that the average daily return is greater than 1%.
What's the Difference Between a T-Test and Z-Test?
Z-tests are closely related to t-tests, but t-tests are best performed when an experiment has a small sample size, less than 30. Also, t-tests assume the standard deviation is unknown, while z-tests assume it is known. If the standard deviation of the population is unknown, but the sample size is greater than or equal to 30, then the assumption of the sample variance equaling the population variance is made while using the z-test.
What Is Central Limit Theorem (CLT)?
In the study of probability theory, the central limit theorem (CLT) states that the distribution of sample approximates a normal distribution (also known as a “bell curve”) as the sample size becomes larger, assuming that all samples are identical in size, and regardless of the population distribution shape. Sample sizes equal to or greater than 30 are considered sufficient for the CLT to predict the characteristics of a population accurately.
What Is a Z-Score?
A z-score, or z-statistic, is a number representing how many standard deviations above or below the mean population the score derived from a z-test is. Essentially, it is a numerical measurement that describes a value's relationship to the mean of a group of values. If a Z-score is 0, it indicates that the data point's score is identical to the mean score. A Z-score of 1.0 would indicate a value that is one standard deviation from the mean. Z-scores may be positive or negative, with a positive value indicating the score is above the mean and a negative score indicating it is below the mean.
Related terms:
Alpha Risk
Alpha risk is the risk in a statistical test of rejecting a null hypothesis when it is actually true. read more
Business Valuation , Methods, & Examples
Business valuation is the process of estimating the value of a business or company. read more
Central Limit Theorem (CLT)
The central limit theorem states that the distribution of sample means approximates a normal distribution as the sample size gets larger. read more
Normal Distribution
Normal distribution is a continuous probability distribution wherein values lie in a symmetrical fashion mostly situated around the mean. read more
Statistical Significance
Statistical significance refers to a result that is not likely to occur randomly but rather is likely to be attributable to a specific cause. read more
T-Test
A t-test is a type of inferential statistic used to determine if there is a significant difference between the means of two groups, which may be related in certain features. read more
T Distribution
A T distribution is a type of probability function that is appropriate for estimating population parameters for small sample sizes or unknown variances. read more
Test
A test is when a stock’s price approaches an established support or resistance level set by the market. read more
Two-Tailed Test
A two-tailed test is the statistical testing of whether a distribution is two-sided and if a sample is greater than or less than a range of values. read more
Z-Score : How to Calculate Z-Score
A Z-Score is a statistical measurement of a score's relationship to the mean in a group of scores. read more