
Nonparametric Method
The nonparametric method refers to a type of statistic that does not make any assumptions about the characteristics of the sample (its parameters) or whether the observed data is quantitative or qualitative. Since nonparametric statistics makes fewer assumptions about the sample data, its application is wider in scope than parametric statistics. This is because nonparametric statistics discard some information that is available in the data, unlike parametric statistics. Nonparametric statistics do not require that the population data meet the assumptions required for parametric statistics. The nonparametric method is a branch of statistics in which the data are not assumed to come from prescribed models that are determined by a small number of parameters.
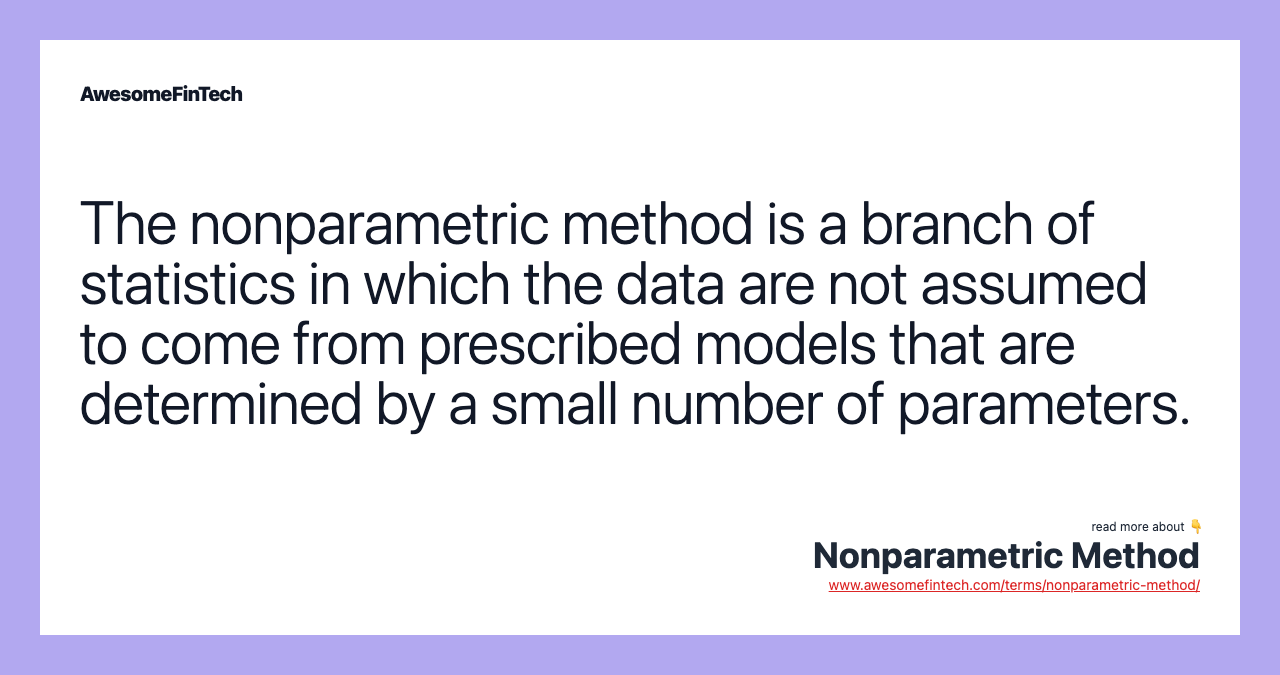
What Is the Nonparametric Method?
The nonparametric method refers to a type of statistic that does not make any assumptions about the characteristics of the sample (its parameters) or whether the observed data is quantitative or qualitative.
Nonparametric statistics can include certain descriptive statistics, statistical models, inference, and statistical tests. The model structure of nonparametric methods is not specified a priori but is instead determined from data.
The term "nonparametric" is not meant to imply that such models completely lack parameters, but rather that the number and nature of the parameters are flexible and not fixed in advance. A histogram is an example of a nonparametric estimate of a probability distribution.
In contrast, well-known statistical methods such as ANOVA, Pearson's correlation, t-test, and others do make assumptions about the data being analyzed. One of the most common parametric assumptions is that population data have a "normal distribution."
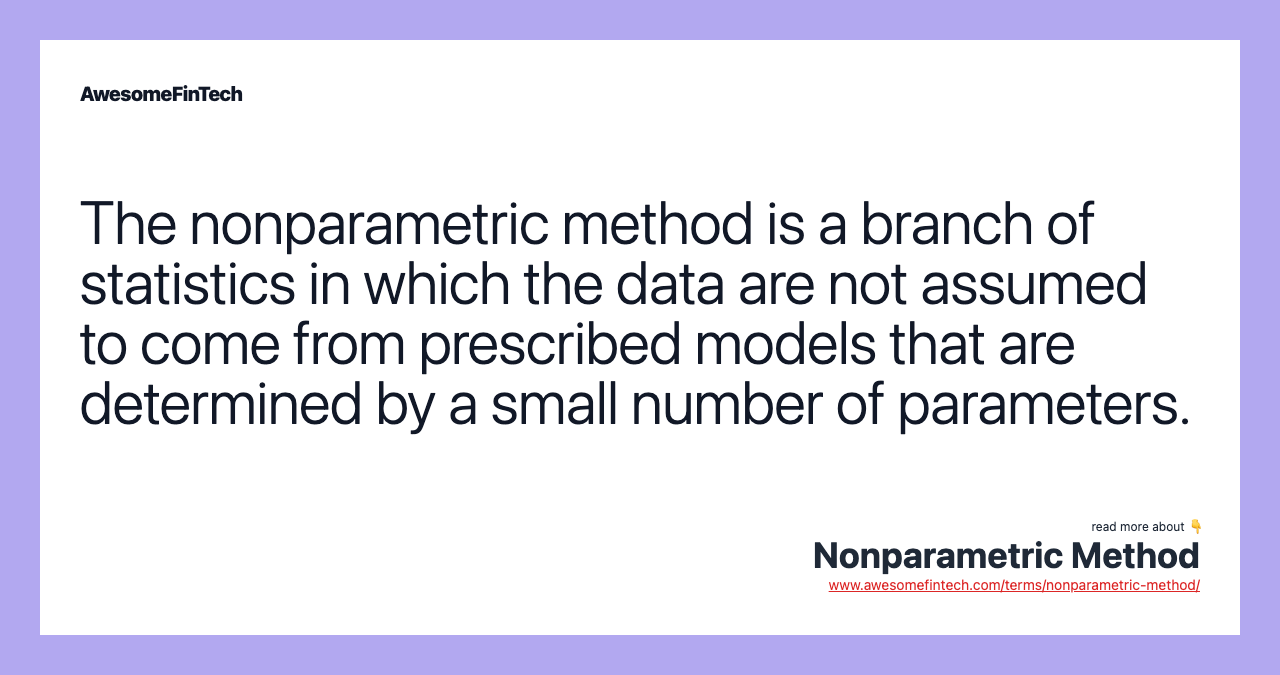
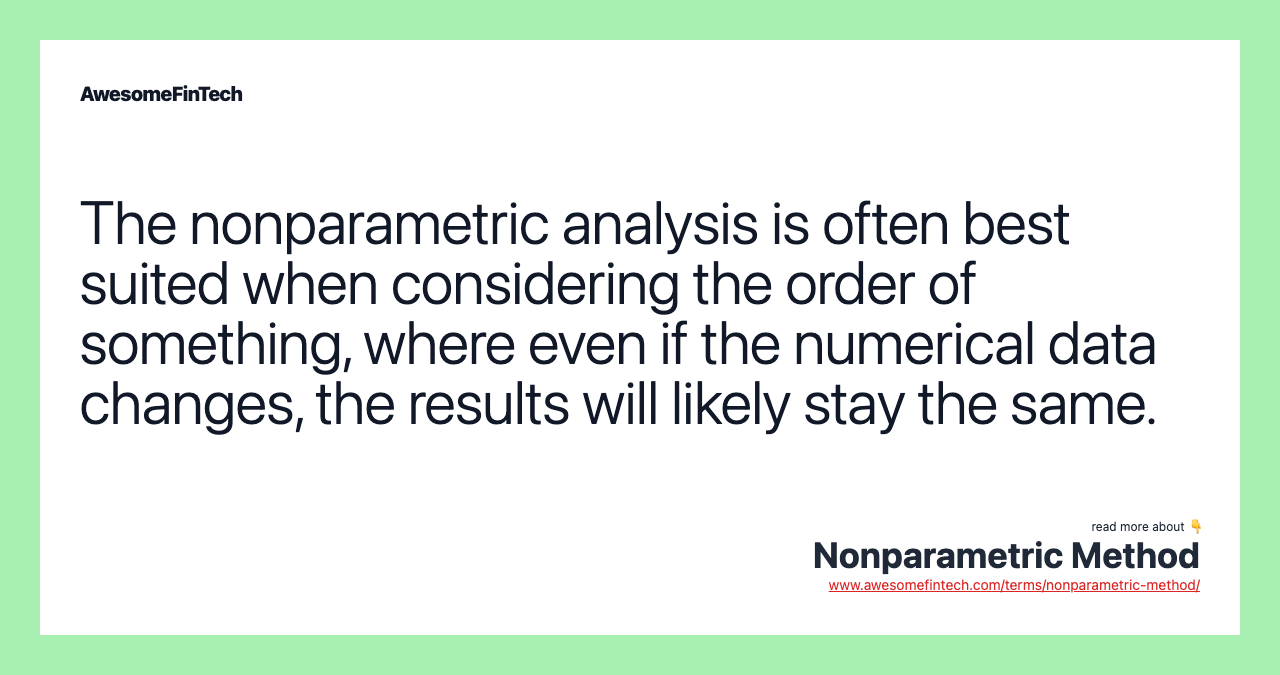
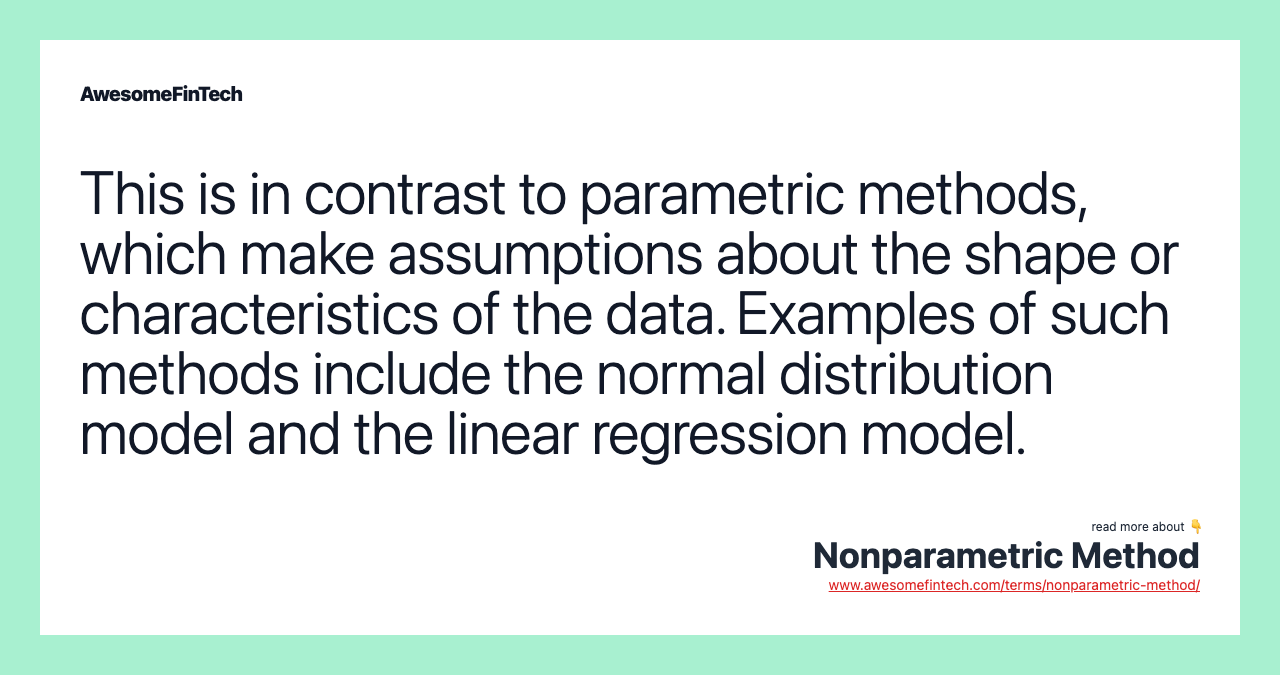
How the Nonparametric Method Works
Parametric and nonparametric methods are often used on different types of data. Parametric statistics generally require interval or ratio data. An example of this type of data is age, income, height, and weight in which the values are continuous and the intervals between values have meaning.
In contrast, nonparametric statistics are typically used on data that nominal or ordinal. Nominal variables are variables for which the values have not quantitative value. Common nominal variables in social science research, for example, include sex, whose possible values are discrete categories, "male" and "female."' Other common nominal variables in social science research are race, marital status, educational level, and employment status (employed versus unemployed).
Ordinal variables are those in which the value suggests some order. An example of an ordinal variable would be if a survey respondent asked, "On a scale of 1 to 5, with 1 being Extremely Dissatisfied and 5 being Extremely Satisfied, how would you rate your experience with the cable company?"
Parametric statistics may too be applied to populations with other known distribution types, however. Nonparametric statistics do not require that the population data meet the assumptions required for parametric statistics. Nonparametric statistics, therefore, fall into a category of statistics sometimes referred to as distribution-free. Often nonparametric methods will be used when the population data has an unknown distribution, or when the sample size is small.
Special Considerations
Although nonparametric statistics have the advantage of having to meet few assumptions, they are less powerful than parametric statistics. This means that they may not show a relationship between two variables when in fact one exists.
Nonparametric statistics have gained appreciation due to their ease of use. As the need for parameters is relieved, the data becomes more applicable to a larger variety of tests. This type of statistics can be used without the mean, sample size, standard deviation, or the estimation of any other related parameters when none of that information is available.
Since nonparametric statistics makes fewer assumptions about the sample data, its application is wider in scope than parametric statistics. In cases where parametric testing is more appropriate, nonparametric methods will be less efficient. This is because nonparametric statistics discard some information that is available in the data, unlike parametric statistics.
Common nonparametric tests include Chi-Square, Wilcoxon rank-sum test, Kruskal-Wallis test, and Spearman's rank-order correlation.
Examples of the Nonparametric Method
Consider a financial analyst who wishes to estimate the value-at-risk (VaR) of an investment. The analyst gathers earnings data from hundreds of similar investments over a similar time horizon. Rather than assume that the earnings follow a normal distribution, she uses the histogram to estimate the distribution nonparametrically. The 5th percentile of this histogram then provides the analyst with a nonparametric estimate of VaR.
For a second example, consider a different researcher who wants to know whether average hours of sleep are linked to how frequently one falls ill. Because many people get sick rarely, if at all, and occasional others get sick far more often than most others, the distribution of illness frequency is clearly non-normal, being right-skewed and outlier-prone.
Thus, rather than use a method that assumes a normal distribution for illness frequency, as is done in classical regression analysis, for example, the researcher decides to use a nonparametric method such as quantile regression analysis.
Related terms:
Analysis of Variance (ANOVA) & Formula
Analysis of variance (ANOVA) is a statistical analysis tool that separates the total variability found within a data set into two components: random and systematic factors. read more
Binomial Distribution
The binomial distribution is a probability distribution that summarizes the likelihood that a value will take one of two independent values. read more
Chi-Square (χ2) Statistic
A chi-square (χ2) statistic is a test that measures how expectations compare to actual observed data (or model results). read more
Descriptive Statistics
Descriptive statistics is a set of brief descriptive coefficients that summarize a given data set representative of an entire or sample population. read more
Nonparametric Statistics: Overview
Nonparametric statistics refer to a statistical method in which the data is not required to fit a normal distribution. Rankings should not change. read more
Normal Distribution
Normal distribution is a continuous probability distribution wherein values lie in a symmetrical fashion mostly situated around the mean. read more
Pearson Coefficient
Pearson coefficient is a type of correlation coefficient that represents the relationship between two variables that are measured on the same interval. read more
Runs Test
Traders use a runs test to determine the randomness of data by revealing any variables that might affect data patterns, such as a stock's price movement. read more
Statistics
Statistics is the collection, description, analysis, and inference of conclusions from quantitative data. read more