
T Distribution
The T distribution, also known as the Student’s t-distribution, is a type of probability distribution that is similar to the normal distribution with its bell shape but has heavier tails.  Image by Sabrina Jiang © Investopedia 2020 When a sample of n observations is taken from a normally distributed population having mean M and standard deviation D, the sample mean, m, and the sample standard deviation, d, will differ from M and D because of the randomness of the sample. A z-score can be calculated with the population standard deviation as Z = (x – M)/D, and this value has the normal distribution with mean 0 and standard deviation 1. Tail heaviness is determined by a parameter of the T distribution called degrees of freedom, with smaller values giving heavier tails, and with higher values making the T distribution resemble a standard normal distribution with a mean of 0, and a standard deviation of 1. The T distribution, also known as the Student’s t-distribution, is a type of probability distribution that is similar to the normal distribution with its bell shape but has heavier tails. the difference between d and D makes the distribution a T distribution with (n - 1) degrees of freedom rather than the normal distribution with mean 0 and standard deviation 1.
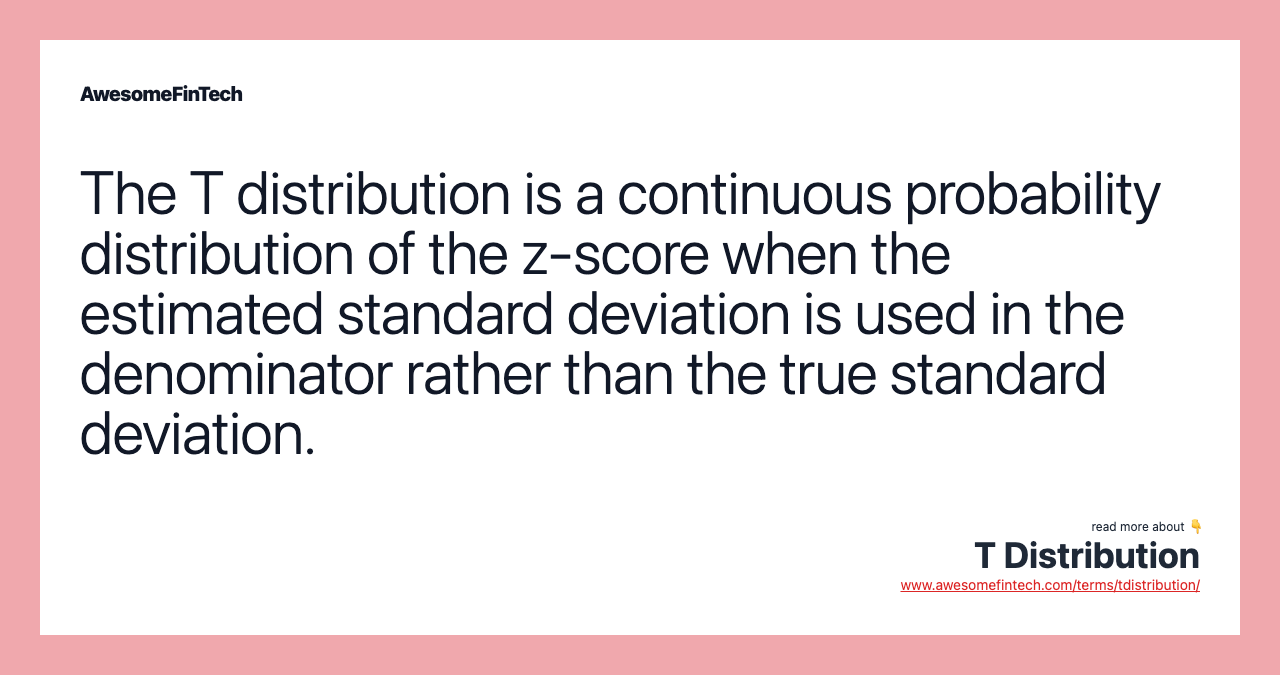
What Is a T Distribution?
The T distribution, also known as the Student’s t-distribution, is a type of probability distribution that is similar to the normal distribution with its bell shape but has heavier tails. T distributions have a greater chance for extreme values than normal distributions, hence the fatter tails.
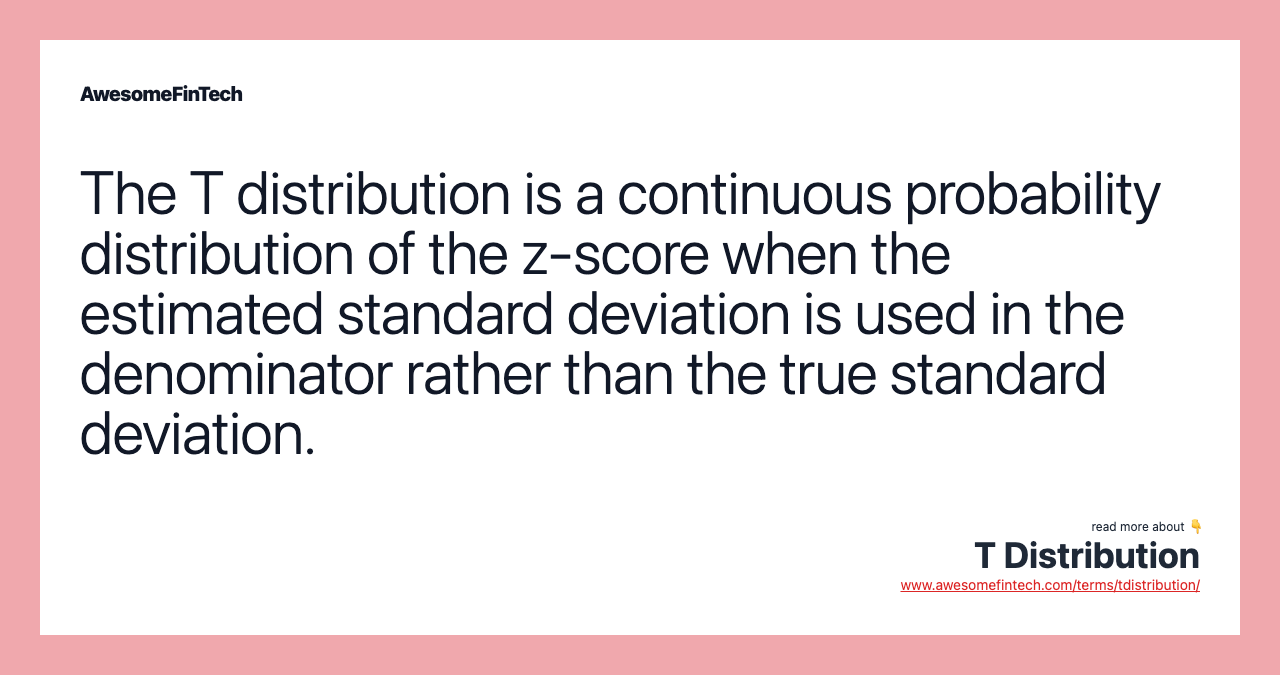
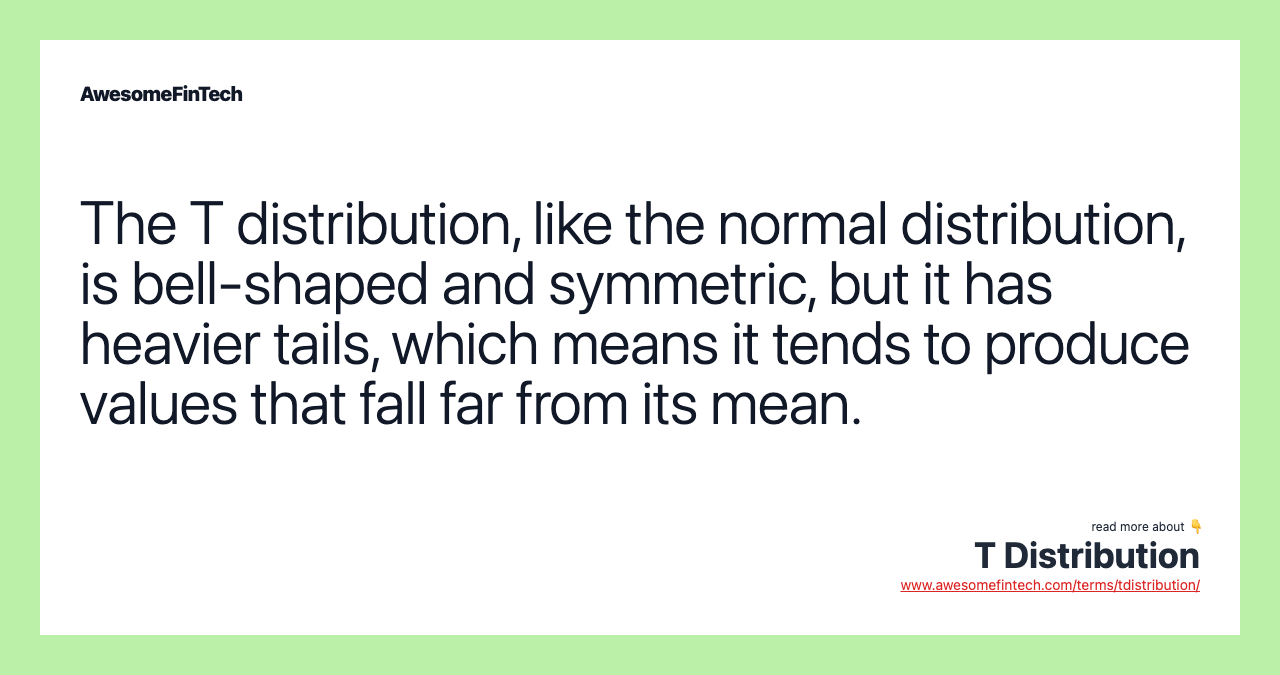
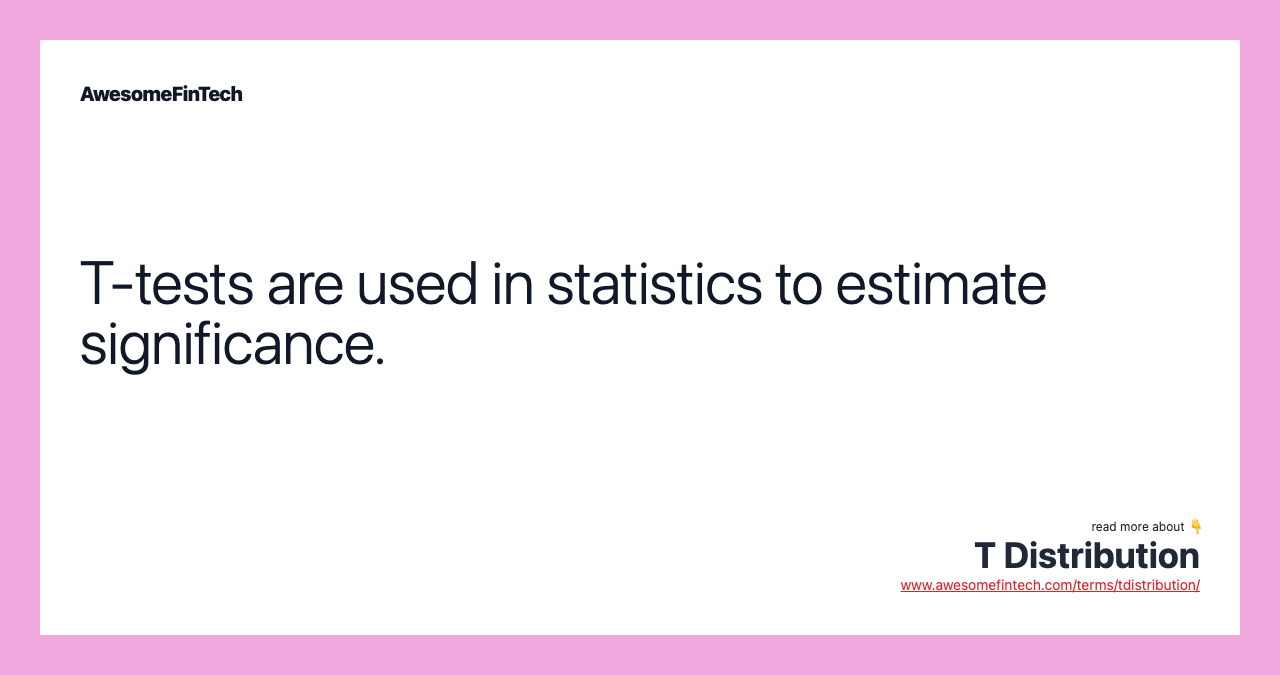
What Does a T Distribution Tell You?
Tail heaviness is determined by a parameter of the T distribution called degrees of freedom, with smaller values giving heavier tails, and with higher values making the T distribution resemble a standard normal distribution with a mean of 0, and a standard deviation of 1. The T distribution is also known as "Student's T Distribution."
Image by Sabrina Jiang © Investopedia 2020
When a sample of n observations is taken from a normally distributed population having mean M and standard deviation D, the sample mean, m, and the sample standard deviation, d, will differ from M and D because of the randomness of the sample.
A z-score can be calculated with the population standard deviation as Z = (x – M)/D, and this value has the normal distribution with mean 0 and standard deviation 1. But when using the estimated standard deviation, a t-score is calculated as T = (m – M)/{d/sqrt(n)}, the difference between d and D makes the distribution a T distribution with (n - 1) degrees of freedom rather than the normal distribution with mean 0 and standard deviation 1.
Example of How to Use a T-Distribution
Take the following example for how t-distributions are put to use in statistical analysis. First, remember that a confidence interval for the mean is a range of values, calculated from the data, meant to capture a “population” mean. This interval is m +- t*d/sqrt(n), where t is a critical value from the T distribution.
For instance, a 95% confidence interval for the mean return of the Dow Jones Industrial Average in the 27 trading days prior to 9/11/2001, is -0.33%, (+/- 2.055) * 1.07 / sqrt(27), giving a (persistent) mean return as some number between -0.75% and +0.09%. The number 2.055, the amount of standard errors to adjust by, is found from the T distribution.
Because the T distribution has fatter tails than a normal distribution, it can be used as a model for financial returns that exhibit excess kurtosis, which will allow for a more realistic calculation of Value at Risk (VaR) in such cases.
The Difference Between a T Distribution and a Normal Distribution
Normal distributions are used when the population distribution is assumed to be normal. The T distribution is similar to the normal distribution, just with fatter tails. Both assume a normally distributed population. T distributions have higher kurtosis than normal distributions. The probability of getting values very far from the mean is larger with a T distribution than a normal distribution.
Limitations of Using a T Distribution
The T distribution can skew exactness relative to the normal distribution. Its shortcoming only arises when there’s a need for perfect normality. The T-distribution should only be used when population standard deviation is not known. If the population standard deviation is known and the sample size is large enough, the normal distribution should be used for better results.
Related terms:
Bell Curve
A bell curve describes the shape of data conforming to a normal distribution. read more
Central Limit Theorem (CLT)
The central limit theorem states that the distribution of sample means approximates a normal distribution as the sample size gets larger. read more
Confidence Interval
A confidence interval, in statistics, refers to the probability that a population parameter will fall between two set values. read more
Degrees of Freedom
Degrees of Freedom refers to the maximum number of logically independent values, which are values that have the freedom to vary, in the data sample. read more
Kurtosis
Kurtosis is a statistical measure used to describe the distribution of observed data around the mean. It is sometimes referred to as the "volatility of volatility." read more
Probability Distribution
A probability distribution is a statistical function that describes possible values and likelihoods that a random variable can take within a given range. read more
T-Test
A t-test is a type of inferential statistic used to determine if there is a significant difference between the means of two groups, which may be related in certain features. read more
Technical Analysis of Stocks and Trends
Technical analysis of stocks and trends is the study of historical market data, including price and volume, to predict future market behavior. read more
Value at Risk (VaR)
Value at risk (VaR) is a statistic that quantifies the level of financial risk within a firm, portfolio, or position over a specific time frame. read more