
Rescaled Range Analysis and Uses
Rescaled range analysis is a statistical technique used to analyze trends in a time series. The rescaled range is calculated by dividing the range (maximum value minus minimum value) of the cumulative mean adjusted data points (sum of each data point minus the mean of the data series) by the standard deviation of the values over the same portion of the time series. Rescaled range analysis looks at a data series and determines the persistence or mean-reverting tendencies within that data. Rescaled range analysis looks at a data series and determines the persistence or mean-reverting tendencies within that data. When H is greater than 0.5, the data is exhibiting a strong long-term trend, and when H is less than 0.5, it is likely to reverse trend over the time frame considered.
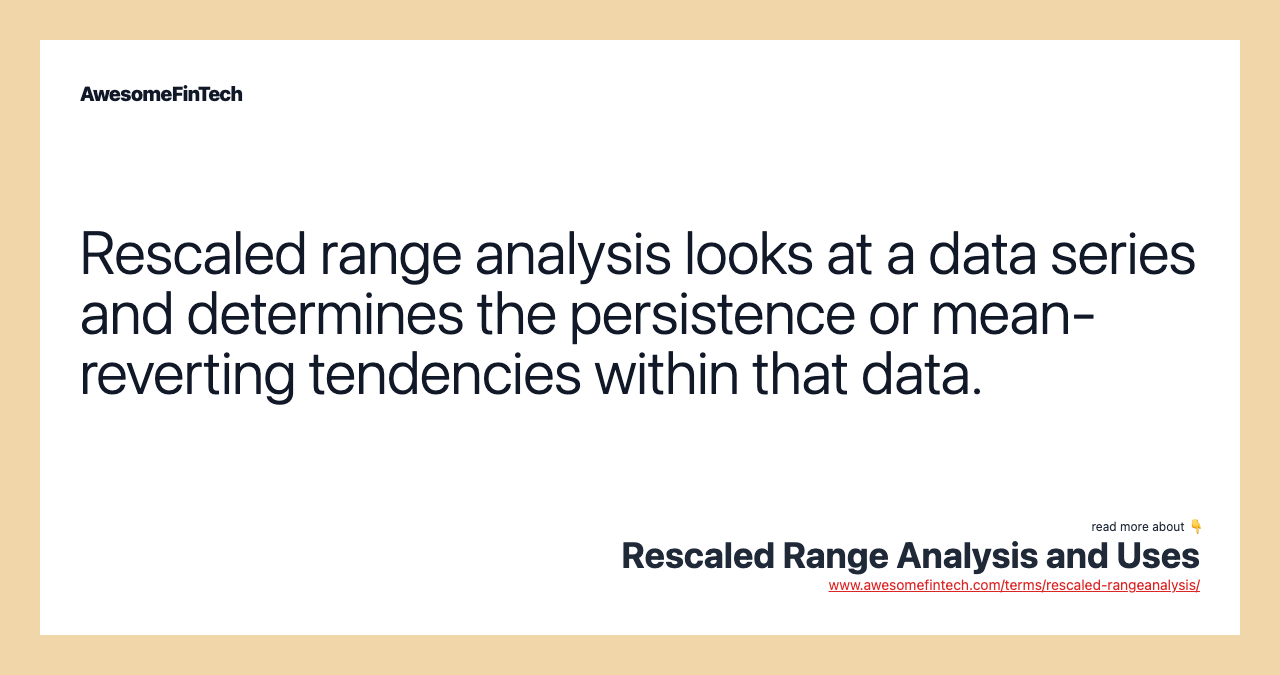
What Is Rescaled Range Analysis?
Rescaled range analysis is a statistical technique used to analyze trends in a time series. It was developed by British hydrologist Harold Edwin Hurst to predict flooding on the Nile river. Investors have used it to look for cycles, patterns, and trends in stock and bond prices that might repeat or reverse in the future.
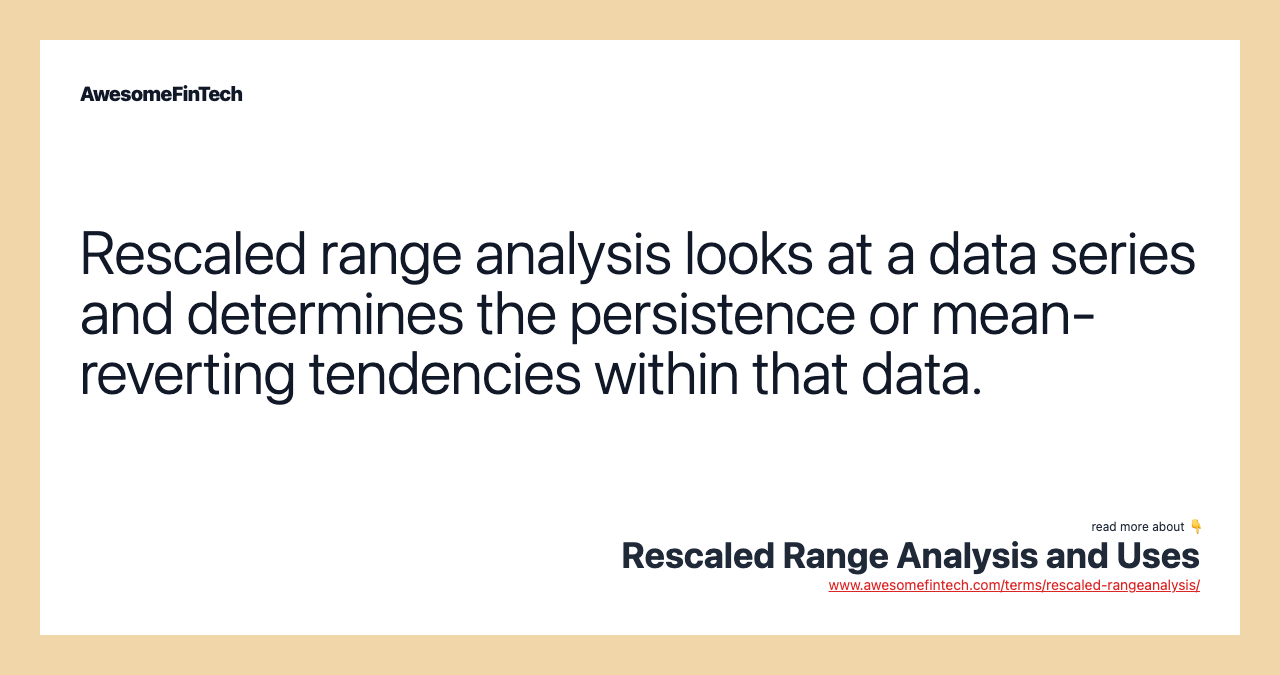
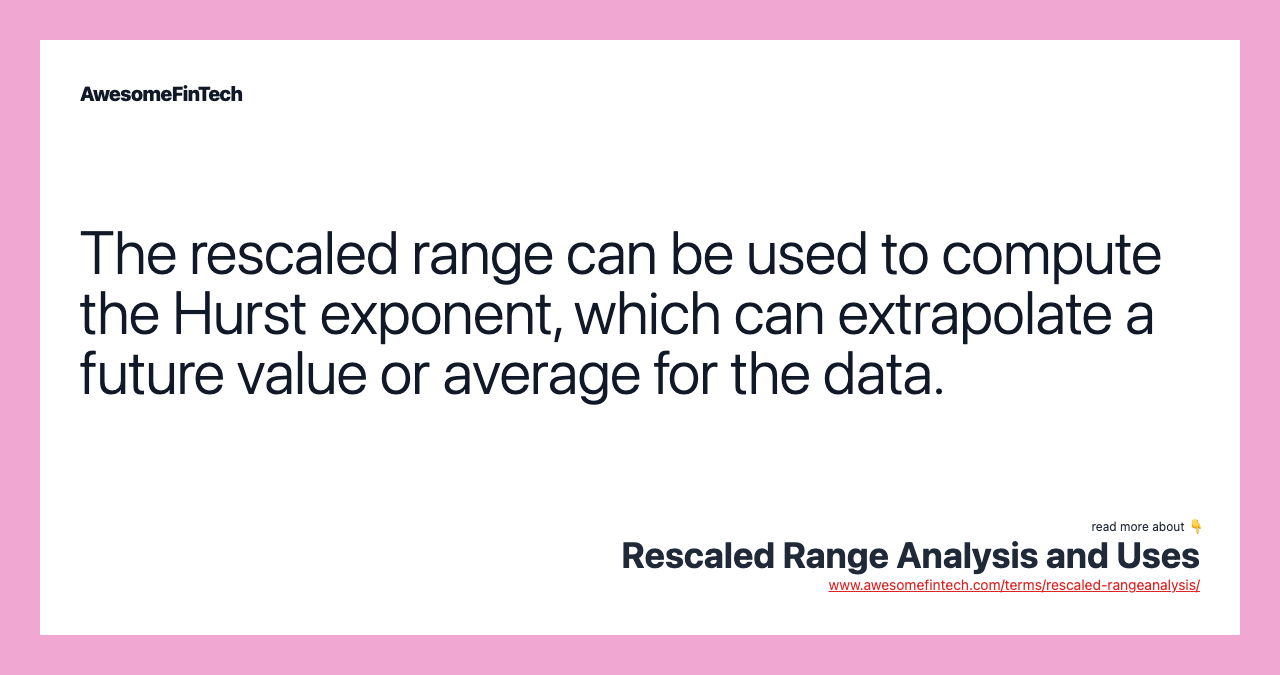
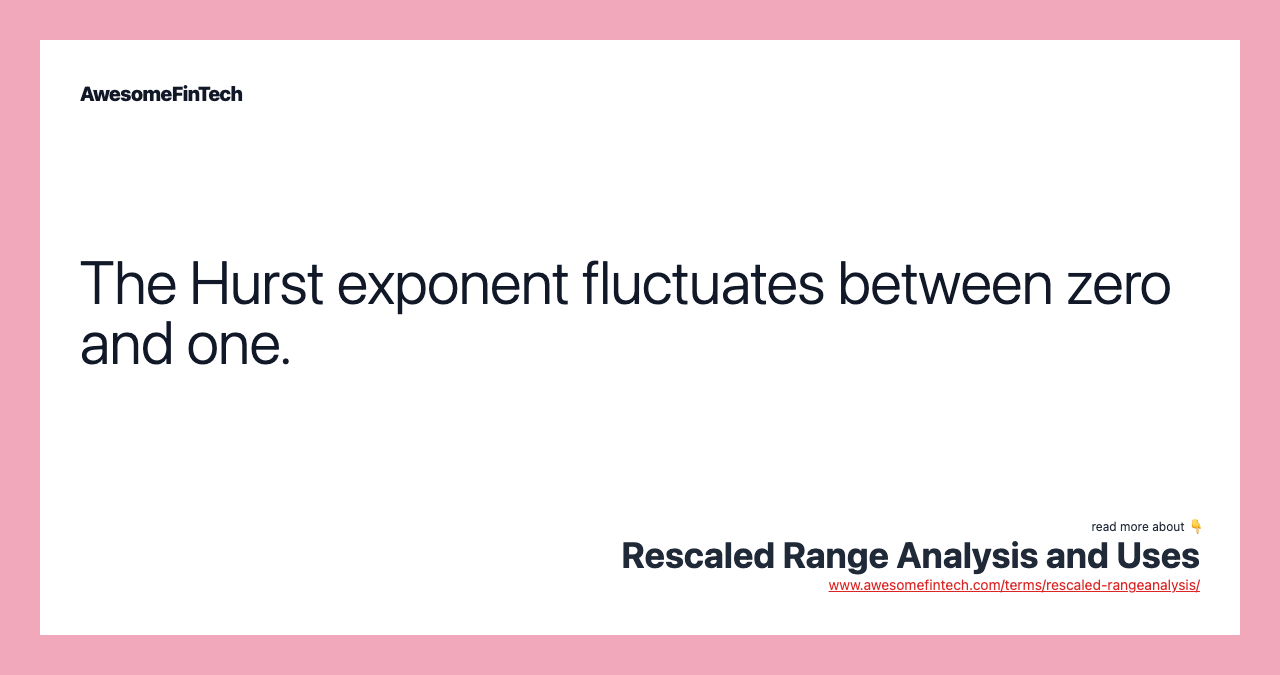
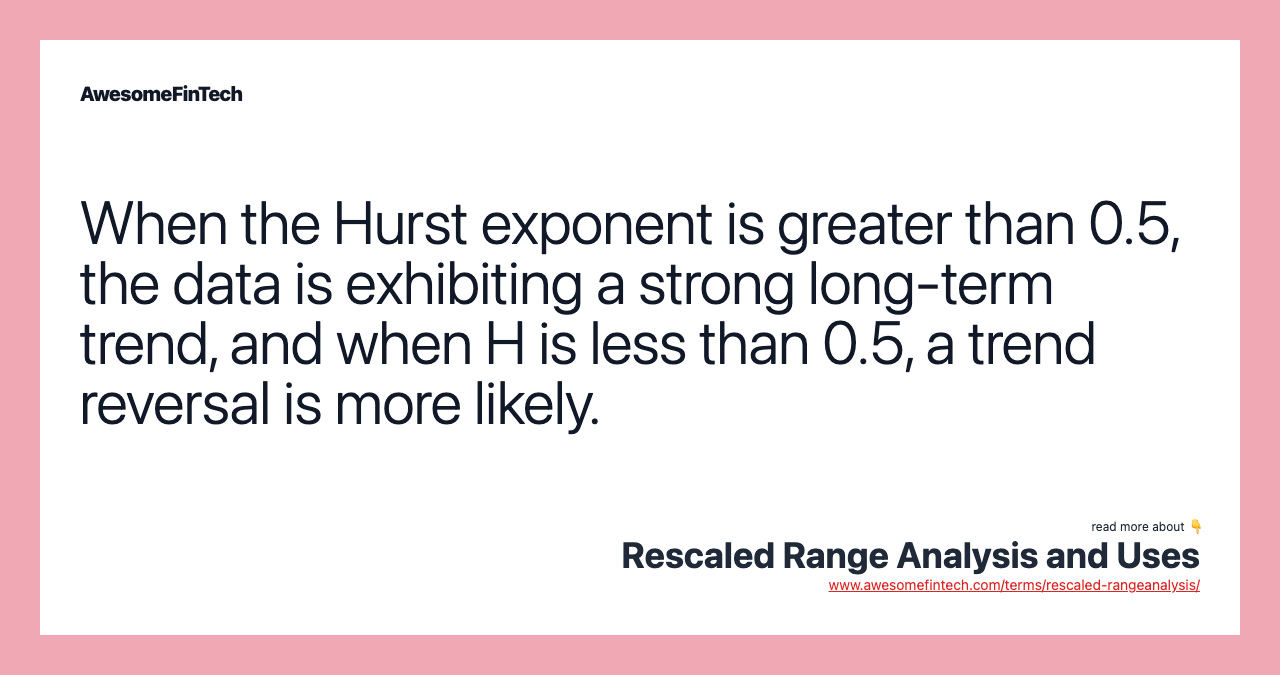
Understanding Rescaled Range Analysis
Rescaled range analysis can be used to detect and evaluate the amount of persistence, randomness, or mean reversion in financial markets time series data. Exchange rates and stock prices do not follow a random walk, or unpredictable path, like they would if price changes were independent of each other. Markets, in other words, are not perfectly efficient, which means there are opportunities for investors to capitalize on.
If a strong trend exists in the data, it will be captured by the Hurst exponent (H exponent), which can also be used to rate mutual funds. The H exponent, which is also known as the index of long-range dependence, can extrapolate a future value or average for the data.
The Hurst exponent ranges between zero and one, and it measures persistence, randomness, or mean reversion. Time series that display a random stochastic process have H exponents close to 0.5. When H is greater than 0.5, the data is exhibiting a strong long-term trend, and when H is less than 0.5, it is likely to reverse trend over the time frame considered.
H exponents below 0.5 are also known as the Joseph effect, in reference to the biblical story of seven years of plenty being followed by seven years of famine. Low values are likely to be followed by high values, or vice versa.
Rescaled Range and the Hurst Exponent
Rescaled range analysis assesses how the variability of times series data changes with the length of the time period being considered. The rescaled range is calculated by dividing the range (maximum value minus minimum value) of the cumulative mean adjusted data points (sum of each data point minus the mean of the data series) by the standard deviation of the values over the same portion of the time series.
As the number of observations in a time series increases, the rescaled range increases. By plotting these increases as the logarithm of R/S versus the logarithm of n, one can determine the slope of this line, which is the Hurst exponent, H.
Examples of How to Use Rescaled Range Analysis
The Hurst exponent can be used in trend trading investment strategies. An investor would be looking for stocks that show strong persistence. These stocks would have an H greater than 0.5. An H less than 0.5 could be paired with technical indicators to spot price reversals. For example, in order to time their investment, a value investor might look for stocks with H less than 0.5 whose prices have been declining for some time.
Mean reversion trading looks to capitalize on extreme changes in the price of a security, based on the assumption that it will revert to its previous state. The H exponent is used by algorithmic traders to speculate on mean-reverting time series strategies like pairs trading, where the spread between two assets is mean-reverting.
The following chart shows a 15-period moving average (MA) of the Hurst Exponent based on the SPDR S&P 500 (SPY) price chart. The MA can be adjusted, with a longer MA smoothing out fluctuations.
For traders wanting to buy during an uptrend in the price, they could look for opportunities where the H is above 0.5 and the price is moving up. Used in this way, the indicator wouldn't necessarily provide trade signals, but it could aid in providing confirmation for other trade signals based on the trend.
Image by Sabrina Jiang © Investopedia 2021
The indicator won't always provide good signals. It is also important to note that high H values when the price is declining are indicating further declines in price, which can make the indicator a bit confusing when first using it.
The Difference Between Rescaled Range Analysis and Regression Analysis
Rescaled range analysis looks at a data series and determines the persistence or mean-reverting tendencies within that data. Linear regression looks at two variables, such as price and time, and finds the midpoint or the line of best fit for the data series. Then, standard deviation channels can be added to show when the security is potentially overbought or oversold based on the data series. Linear regression is part of the larger field of regression analysis.
Limitations of Rescaled Range Analysis
For trading purposes, a rescaled range is the adjusted range divided by the standard deviation. These calculations are based on past data and aren't inherently predictive. It is up to the trader to interpret the information the rescaled range or Hurst exponent is providing.
For trading purposes, the Hurst indicator, which is derived from the rescaled range, may work sometimes, but it doesn't work all the time. A strong price trend could be reversed sharply, which the indicator did not foresee. Reversals signaled by the indicator may also not develop.
Related terms:
Algorithmic Trading
Algorithmic trading is a system that utilizes very advanced mathematical models for making transaction decisions in the financial markets. read more
Autoregressive Integrated Moving Average (ARIMA)
An autoregressive integrated moving average (ARIMA) is a statistical analysis model that leverages time series data to forecast future trends. read more
Joseph Effect
The Joseph Effect is the concept that persistence over time occurs within otherwise random movements and can be used to predict future prosperity. read more
Kondratiev Wave
Kondratiev Wave is a long-term economic cycle, believed to result from technological innovation, that produces a long period of economic prosperity. read more
Mean Reversion
Mean reversion is a financial theory positing that asset prices and historical returns eventually revert to their long-term mean or average level. read more
Morningstar Risk Rating
Morningstar risk ratings assess one of five levels of risk for mutual funds and ETFs to give investors a quick idea of their portfolio suitability. read more
Moving Average (MA)
A moving average (MA) is a technical analysis indicator that helps smooth out price action by filtering out the “noise” from random price fluctuations. read more
Polynomial Trending
Polynomial trending occurs in large data sets containing many fluctuations and describes a curved or broken pattern from a straight linear trend. read more
Random Walk Index and Uses
The random walk index compares a security's price movements to a random sampling to determine if it's engaged in a statistically significant trend. read more
Random Walk Theory and Example
Random walk theory suggests that changes in stock prices have the same distribution and are independent of each other. read more